Unleashing AI from the Cloud: The Dawn of Edge, Decentralized, and Neuromorphic Intelligence
- Aki Kakko
- 7 hours ago
- 5 min read
For years, Artificial Intelligence has largely resided in the cloud. Powerful data centers, packed with high-performance processors, served as the brains behind our digital assistants, recommendation engines, and complex analytics. While immensely successful, this centralized model faces growing limitations: latency issues for real-time applications, massive bandwidth requirements, significant energy consumption, privacy concerns as data funnels to a single point, and vulnerability to single points of failure. The future of AI is moving closer to where the data is generated and where decisions need to be made – at the "edge" of the network and across distributed systems. This shift towards Edge AI and Decentralized AI, potentially powered by revolutionary compute paradigms like neuromorphic chips, promises a more responsive, private, robust, and sustainable AI ecosystem. For AI researchers setting up a lab today, this convergence point represents a fertile ground for groundbreaking contributions.
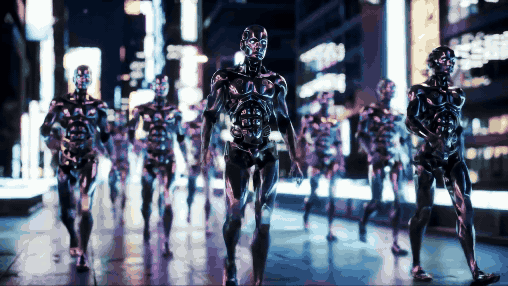
Edge AI: Intelligence on the Device
Edge AI brings AI computation directly onto local devices – smartphones, smart cameras, industrial sensors, autonomous vehicles, wearables, and even tiny microcontrollers in everyday objects. The primary drivers are clear:
Low Latency: Decisions are made instantly without round trips to the cloud, critical for applications like autonomous driving or real-time anomaly detection in manufacturing.
Reduced Bandwidth: Only necessary data (or decisions) needs to be transmitted, saving costs and enabling operation in areas with poor connectivity.
Enhanced Privacy: Sensitive data can be processed locally, minimizing the risk of breaches during transit or storage in the cloud.
Offline Capability: Edge AI can function even when disconnected from the internet.
However, deploying complex AI models on edge devices is challenging due to severe constraints on compute power, memory, and energy. Current Edge AI research focuses heavily on:
Model Compression: Developing techniques like pruning (removing unnecessary connections), quantization (reducing the precision of weights), and knowledge distillation (training a small model to mimic a larger one) to shrink models while preserving accuracy.
Efficient Architectures: Designing neural network models specifically built for efficiency, with fewer parameters and operations.
Hardware-Aware Optimization: Tailoring models and inference engines to run optimally on specialized edge AI accelerators (NPUs, TPUs, etc.).
Decentralized AI: The Power of the Collective
Decentralized AI takes the distribution concept further, distributing not just the inference but also potentially the training process and data storage across multiple nodes or devices, often without relying on a single central server for everything. The most prominent example is Federated Learning, where models are trained locally on devices using their private data, and only model updates (like weight changes) are aggregated centrally or in a decentralized manner.
The advantages of Decentralized AI are compelling:
Privacy by Design: Data never leaves the device, addressing a major barrier for using sensitive information (e.g., healthcare, personal data).
Increased Robustness: No single point of failure; the system can continue to function even if some nodes go offline.
Scalability: The computational burden is distributed across many devices.
Reduced Communication (often): Only model parameters or updates are shared, not raw data.
Research in Decentralized AI is grappling with complex issues:
Handling Heterogeneity: Devices have vastly different capabilities, network conditions vary wildly, and data distribution is often non-uniform (non-IID data).
Communication Efficiency: While less data is sent, coordinating potentially millions of devices and aggregating updates efficiently is a challenge.
Security and Trust: Protecting against malicious participants who might send poisoned updates (Byzantine attacks) or try to infer private data from shared parameters.
Orchestration and Management: How to effectively deploy, monitor, and update models across a dynamic, decentralized network.
The Powerful Synergy: Edge and Decentralized AI
It's clear that Edge AI and Decentralized AI are not separate paths but rather deeply intertwined. Edge devices are the natural endpoints for a decentralized AI system. Federated learning, for instance, is an ideal training paradigm for models that will ultimately reside and run inferences on edge devices.
A research lab focused on the future must operate at the intersection of these two fields. This involves:
Developing federated learning algorithms optimized for the computational and memory constraints of edge devices.
Researching privacy-preserving techniques (like Differential Privacy or Secure Multi-Party Computation) that can be implemented efficiently on resource-limited hardware.
Creating decentralized model management systems tailored for intermittent connectivity and diverse edge hardware.
Building algorithms for on-device, federated adaptation and personalization of models.
Looking Ahead: The Neuromorphic Computing Paradigm
While current Edge and Decentralized AI largely relies on optimizing traditional deep learning models for conventional silicon (CPUs, GPUs, NPUs), the future holds the potential for a fundamental shift in computing hardware itself. This is where Neuromorphic Computing enters the picture. Inspired by the structure and function of the human brain, neuromorphic chips operate differently from traditional Von Neumann architectures. Instead of discrete memory and processing units clocked synchronously, they feature interconnected "neurons" and "synapses" that process information via asynchronous "spikes" (electrical pulses).
Why is this revolutionary for Edge and Decentralized AI?
Extreme Energy Efficiency: Spiking Neural Networks (SNNs) running on neuromorphic hardware are event-driven – computation only happens when a "spike" occurs. This is orders of magnitude more energy-efficient for certain tasks (like processing sensor data) compared to traditional chips that consume power constantly regardless of input activity. This is critical for battery-powered edge devices.
Low Latency: Information propagates through the network rapidly via spikes, potentially enabling ultra-fast responses.
Parallelism: The highly parallel nature of neuromorphic architectures is naturally suited for processing the constant streams of data from edge sensors.
Potential for On-Chip Learning: Some neuromorphic designs are exploring biologically plausible mechanisms for local, on-chip learning and adaptation, aligning perfectly with the goals of on-device training.
Neuromorphic computing is still an emerging field. Key research challenges include:
Training Algorithms: Developing effective and scalable training methods for SNNs is significantly different and often more complex than for traditional ANNs.
Hardware Development: Designing and manufacturing robust, scalable, and programmable neuromorphic chips.
Software Ecosystem: Creating user-friendly software frameworks, compilers, and tools to map complex AI tasks onto spiking hardware.
Bridging the Gap: Identifying the specific tasks and applications where the unique advantages of neuromorphic computing truly outperform optimized conventional hardware on the edge (e.g., audio processing, event-based vision, sensor fusion).
A Future Shaped by Distributed Intelligence
A research lab focusing on the future of Edge and Decentralized AI, while also exploring the potential of neuromorphic computing, is positioning itself to tackle the most significant challenges and opportunities in AI today. The convergence of these fields promises a future where AI is not confined to distant data centers but is ubiquitous, embedded in our environment, respecting our privacy, operating reliably even offline, and doing so with unprecedented energy efficiency. From smart cities and autonomous systems to personalized healthcare and truly intelligent IoT devices, the impact of this distributed, efficient intelligence will be transformative. The path forward requires deep research across ML algorithms, distributed systems, security, privacy, and revolutionary hardware design, paving the way for a more intelligent, resilient, and human-centric world.
Comentarios