Financial volatility forecasting is a fundamental tool in the kit of every savvy investor. It is a statistical measure of the dispersion of returns for a given security or market index, capturing the degree of variation in trading prices. In simpler terms, volatility is about the intensity of price fluctuations. High volatility means that a security's value can potentially be spread out over a large range of values, which can be an indicator of market uncertainty. Low volatility, on the other hand, signifies that a security's value does not fluctuate dramatically but changes at a steady pace over a period. Understanding and predicting financial volatility can help investors manage risk, optimize portfolios, and identify investment opportunities.
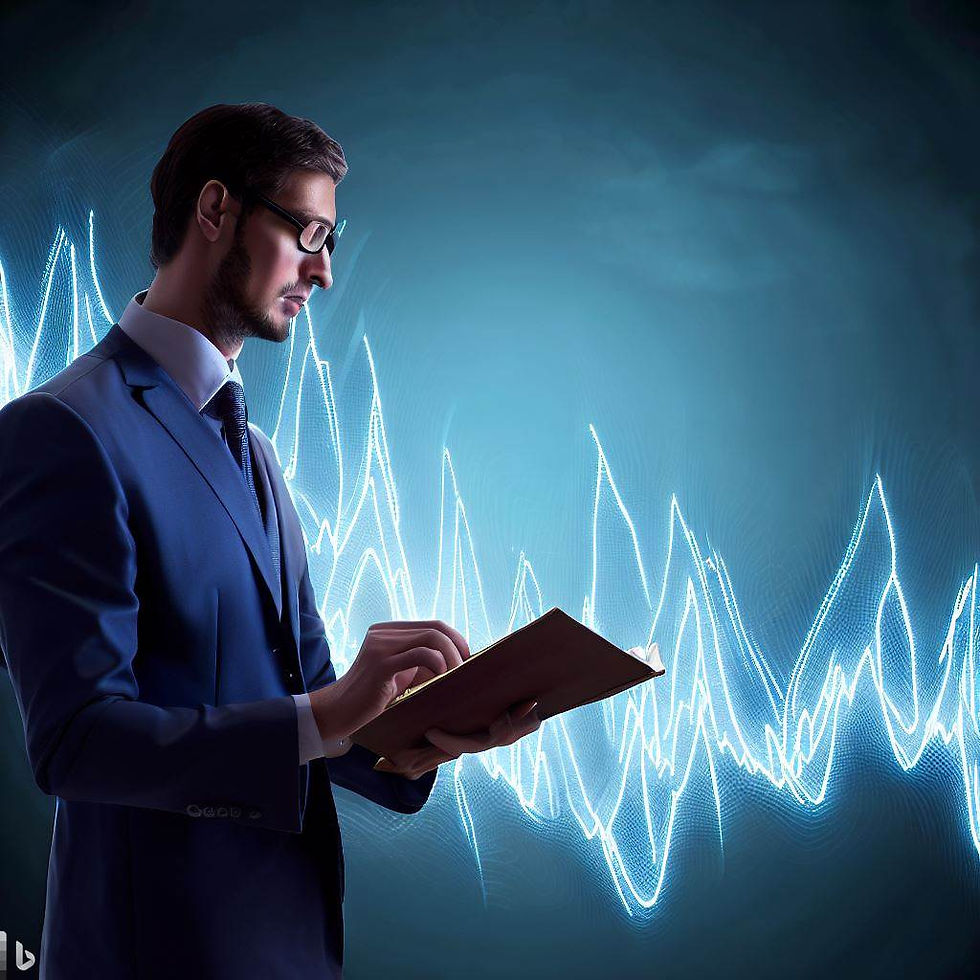
Methods of Financial Volatility Forecasting
Historical Volatility: The most straightforward approach is to compute the standard deviation of past returns. This method uses past returns data to estimate future volatility. For example, an investor can take the standard deviation of a stock's daily return over the last 30 days as a simple estimate of that stock's volatility.
Implied Volatility: Implied volatility is derived from the price of an option and represents the market's expectation of future volatility. It's a forward-looking measure unlike historical volatility. For instance, a high implied volatility will result in a higher option price, all else being equal. This is because when a stock is expected to be more volatile, there's a greater chance it could make a large move and consequently bring higher payoff to the option holder.
Stochastic Volatility Models: These models assume that volatility itself is a random process, distinct from the underlying financial instrument's price. The Heston model is a commonly used stochastic volatility model that allows for a volatility skew, which provides a more realistic representation of market dynamics.
GARCH Models: The Generalized Autoregressive Conditional Heteroskedasticity (GARCH) model is another commonly used tool for forecasting volatility. The GARCH model allows the volatility to change over time as a function of both past errors and past volatility. This model is particularly suited for financial markets where volatility clustering is often observed, i.e., periods of high volatility are often followed by high volatility and vice versa.
Machine Learning and AI: With the advent of big data and advanced computational techniques, machine learning algorithms and AI are increasingly being used to forecast volatility. These techniques can handle a large amount of data, and they can capture complex, non-linear relationships in the data that traditional models might miss.
Applications of Financial Volatility Forecasting
Risk Management: Forecasting volatility is crucial for risk management. For instance, Value-at-Risk (VaR) models, used to measure the risk of loss for investments, require an estimate of future volatility.
Option Pricing: The Black-Scholes option pricing model uses volatility as an input to determine the price of an option. More advanced models such as the Heston model mentioned above, use a stochastic volatility process for option pricing.
Portfolio Optimization: Modern Portfolio Theory (MPT) relies on an estimate of the volatility (or risk) of assets to determine the optimal asset allocation in a portfolio.
Trading Strategies: Many trading strategies such as straddles or strangles are dependent on predicting volatility accurately. Traders who expect high volatility will use different strategies compared to those who expect low volatility.
Financial volatility forecasting is an integral part of financial markets, influencing decision-making processes in portfolio management, option pricing, and risk management. Although the forecasting methods vary in their complexity and assumptions, understanding their underlying principles can empower investors to manage risk more effectively and to optimize returns. Despite the uncertainty and challenges inherent in forecasting, by harnessing the power of these models and techniques, investors can significantly enhance their decision-making ability in the complex world of investing.
Comments