In the rapidly evolving domain of Artificial Intelligence, Transfer Learning has emerged as a pivotal technique, enabling machines to learn faster and more efficiently. For investors, understanding Transfer Learning can offer insights into the potential of startups, the efficiency of AI systems, and the overall trajectory of the AI industry. In this article, we'll delve into what Transfer Learning is, its significance, and its potential advantages for businesses.
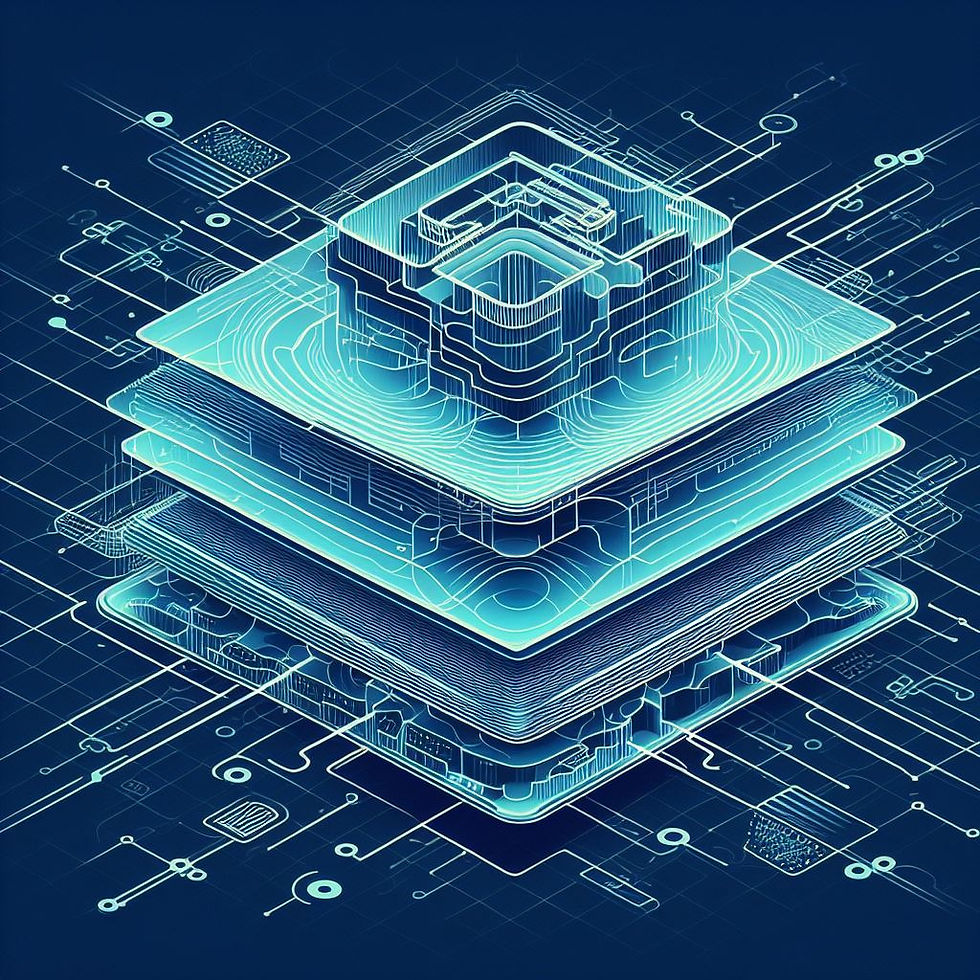
What is Transfer Learning?
Transfer Learning is a machine learning technique where a model developed for a certain task is reused as the starting point for a model on a second task. Instead of starting from scratch, Transfer Learning leverages the knowledge gained from previously solved problems to expedite the learning process for new tasks. Example: Imagine you're an expert in identifying various breeds of dogs. Now, if you're given the task of differentiating between cat breeds, you wouldn't start from zero. You'd use your prior knowledge about animal features, fur patterns, and sizes, and apply that to cats. This is similar to Transfer Learning in AI.
Significance in AI
Data Efficiency: Acquiring labeled data for training can be expensive and time-consuming. Transfer Learning enables models to achieve considerable accuracy with less data.
Training Time: Training deep learning models from scratch requires substantial computational resources and time. By leveraging pre-trained models, the training time for new tasks can be significantly reduced.
Generalization: Models trained on diverse datasets often generalize better to new tasks.
Real-world Examples
Image Recognition: Companies like Google and Facebook use Transfer Learning to fine-tune models for specific tasks. For instance, a model trained on millions of generic images can be fine-tuned with a smaller set of medical images to detect diseases.
Natural Language Processing (NLP): OpenAI's GPT series can be fine-tuned on specific text domains. For instance, a general language model can be specialized to generate legal documents or medical prescriptions.
Voice Assistants: Companies like Amazon and Apple employ Transfer Learning to adapt their generic voice recognition systems to understand user-specific accents and pronunciations.
Challenges of Transfer Learning
While Transfer Learning has paved the way for various advancements in AI, it comes with its own set of challenges:
Domain Discrepancy: For Transfer Learning to be effective, there needs to be some commonality between the source and target tasks. If the two domains are very different, the knowledge transfer might not be beneficial and can even be detrimental.
Overfitting: There’s a risk of overfitting when fine-tuning pre-trained models on small datasets. This means the model might perform exceptionally well on the training data but fail to generalize to new, unseen data.
Complexity: In some scenarios, using a massive pre-trained model might be overkill. It might bring unnecessary computational overhead when a simpler model could have sufficed.
Lack of Interpretability: Transfer Learning, especially in deep learning, can make it harder to interpret how decisions are made, leading to trust issues, especially in critical applications like healthcare.
Future of Transfer Learning
The technique, while still maturing, holds immense promise:
Few-shot and Zero-shot Learning: These are emerging subfields where models are trained to understand tasks with very few examples or even none. Transfer Learning is foundational to these areas, and breakthroughs here can redefine the boundaries of what's possible with AI.
Cross-modal Transfer Learning: Transferring knowledge between different types of data, like text to images or vice versa, can open up fascinating applications, such as generating descriptive text for pictures or visualizing abstract concepts from text.
Continuous Learning: In real-world applications, data is ever-evolving. Models need to adapt continuously. Transfer Learning can play a pivotal role in systems that learn perpetually from new data without forgetting prior knowledge.
Investment Implications
Evaluating Startup Potential: Startups that employ Transfer Learning effectively can: Minimize infrastructure costs. Reduce data acquisition challenges. Quicken product launches.
Competitive Edge: Companies leveraging Transfer Learning can often adapt faster to changing market needs, offering products that are both efficient and versatile.
Intellectual Property: Pre-trained models can become valuable IP assets. Companies owning widely-adopted pre-trained models can license them or offer fine-tuning as a service, opening new revenue streams.
Tips for Investors
Look Beyond the Hype: While Transfer Learning is powerful, it's essential to evaluate if businesses are using it where it genuinely adds value or merely as a buzzword.
Invest in Education: The landscape of AI is vast and ever-changing. Regularly update your knowledge, attend seminars, and consider consulting with AI experts to make informed decisions.
Diversify: While putting your stakes in AI-driven businesses, consider diversifying across various applications of Transfer Learning to mitigate risks associated with specific domains or technologies.
Transfer Learning, without a doubt, has cemented its position as a cornerstone technique in AI. As we stand at the brink of countless AI-driven innovations, savvy investors who comprehend its implications, benefits, and challenges are better poised to capitalize on the opportunities it presents. As with any investment, thorough research, understanding the underlying technology, and consulting with experts will always be the hallmarks of successful decision-making.
Comentarios