The Temporal Tapestry: The Nexus of Language, Cognition, Time, and AI
- Aki Kakko
- 9 hours ago
- 31 min read
Time, often conceived as a fundamental, objective dimension of the physical universe ticking forward with metronomic regularity, reveals itself upon closer inspection to be a profoundly subjective and cognitively constructed aspect of human experience. While clocks measure duration with impersonal precision, our internal sense of time stretches and compresses, influenced by emotion, attention, memory, and perhaps most intriguingly, the very language we use to describe it. Human experience of duration exhibits systematic, context-dependent deviations from clock time; time unfolds differently during moments of intense focus compared to periods of boredom, at work versus on holiday. This constructed nature of temporal perception, far from being a mere cognitive quirk, lies at the heart of how we understand causality, sequence events, plan for the future, and build coherent narratives of our lives. This article looks into the intricate nexus connecting language, the cognitive construction of time, and the burgeoning field of Artificial Intelligence. The central proposition is that understanding the diverse ways humans perceive, conceptualize, and linguistically frame time offers critical insights into the mechanisms of human intelligence itself. Furthermore, this understanding presents both formidable challenges and significant opportunities for the development of AI systems capable of more sophisticated temporal reasoning and interaction.
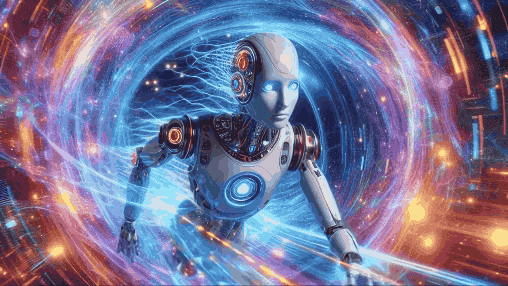
The challenge for AI is substantial. Current leading AI architectures, particularly those successful in natural language processing and sequential data analysis like Recurrent Neural Networks (RNNs) and Transformers, primarily process information based on its order in a sequence. While effective for many tasks, this implicitly reinforces a linear, step-by-step view of time. Can AI transcend these linear representations to model the more complex, varied, and sometimes non-linear temporal structures evident in human cognition and language? What can AI developers learn from the Aymara speaker who faces the past, the Mandarin speaker who conceptualizes time vertically, or the Kuuk Thaayorre speaker whose temporal map is etched onto the landscape by the sun's path? How does the brain's own predictive machinery construct our subjective temporal reality, and can these neurobiological principles inform AI design? This article navigates these questions through an interdisciplinary lens. It begins by examining the concept of linguistic relativity, exploring how language structures might shape our perception and cognition of time. It then presents compelling examples from diverse languages that illustrate radically different conceptualizations of temporality. Following this, the neuroscientific perspective is explored, detailing how the brain actively constructs our experience of time through mechanisms like sensory integration and predictive processing. The discussion then ventures into more theoretical territory, considering philosophical concepts of non-linear time, such as the block universe, and their potential relevance. Subsequently, the article analyzes how current AI models handle time and investigates theoretical and emerging research into alternative, potentially non-linear, AI temporal processing, including the crucial role of causal inference. Finally, it synthesizes these findings, discussing the implications of richer temporal capabilities for AI and exploring how a deeper understanding of human temporal cognition can serve as a blueprint for creating more intelligent, adaptive, and perhaps even more human-like artificial systems.
Linguistic Relativity: How Language Molds Our Temporal World
The notion that the language we speak influences how we think and perceive the world, known as the Sapir-Whorf hypothesis or linguistic relativity, provides a crucial starting point for understanding the connection between language and time.
While the strong version of this hypothesis, linguistic determinism – the idea that language dictates thought and limits cognitive capabilities – has been largely discredited, the weaker version, linguistic relativity – suggesting that language influences or shapes cognitive processes – continues to garner empirical support and stimulate debate.
The hypothesis emerged from the work of linguistic anthropologists like Franz Boas, Edward Sapir, and Benjamin Lee Whorf. Boas emphasized studying diverse languages, particularly Native American languages, to understand the range of linguistic structures and cultural perspectives. Sapir expanded on this, exploring the link between language and thought and introducing the idea that language habits unconsciously shape our perception of the "real world". Whorf, Sapir's student, pushed the idea further, arguing based on his studies of languages like Hopi that linguistic structures directly affect how speakers perceive reality, including fundamental concepts like time. Whorf famously contrasted the Hopi language, which he argued lacked grammatical markers equivalent to English past, present, and future tenses and conceptualized time cyclically, with the linear, quantifiable view he saw embedded in English grammar. His analysis suggested that language doesn't merely reflect reality but actively molds it.
Linguistic relativity proposes several mechanisms through which language might exert its influence. The lexicon, or vocabulary, provides categories for organizing experience. The availability or lack of specific words can make it easier or harder to talk about, attend to, or perhaps even perceive certain distinctions. Research on color perception across languages provides a classic example: languages differ in their number and boundaries of basic color terms, and studies have shown correlations between these linguistic categories and performance on color memory and discrimination tasks, suggesting language influences how colors are perceived and grouped. Similarly, grammatical structures, such as tense systems, aspect markers, or the spatial metaphors used to talk about time, can establish habitual ways of thinking. If a language consistently uses spatial terms like "ahead" for the future and "behind" for the past, this may reinforce a linear, forward-moving mental model of time. Whorf's observation about English speakers treating 'empty' gasoline drums (full of vapor) as inert based solely on their liquid content, leading to dangerous behavior like smoking nearby, illustrates how linguistic categorization can shape real-world actions. Further evidence comes from studies showing that the grammatical gender assigned to objects in languages like Spanish and German influences speakers' descriptions of those objects, attributing masculine or feminine qualities based on the linguistic category rather than inherent features.
However, the relationship is complex. Language is also a human creation, a tool honed to suit our needs, raising a "chicken and egg" question about whether language shapes thought or thought shapes language. Furthermore, the influence of language may not be absolute but rather probabilistic and context-dependent. Some research suggests that linguistic category effects, like those seen in color perception, are strongest when perceptual information is ambiguous, uncertain, or needs to be recalled from memory. A computational model proposes that under such uncertainty (caused by perceptual noise or memory decay), the brain combines fine-grained perceptual representation with language-specific category information, biasing the reconstruction towards the category prototype. This implies that language categories might act as cognitive anchors or priors, helping to stabilize perception and memory when sensory input is degraded or incomplete. Thus, language may not rigidly determine what we perceive in the moment but can significantly shape how we categorize, remember, and reason about our experiences, particularly under conditions of cognitive load or uncertainty. It acts less like a prison for thought and more like cognitive scaffolding, providing structures and categories that make certain ways of thinking – including thinking about time – more natural, accessible, or habitual for its speakers.
A Spectrum of Time: Cross-Linguistic Perspectives
The way time is conceptualized and linguistically encoded varies dramatically across the world's languages, challenging the assumption that the linear, forward-moving "arrow of time" prevalent in many Western cultures is a cognitive universal. Examining languages like Aymara, Mandarin, and Kuuk Thaayorre reveals alternative, yet equally coherent, frameworks for understanding temporality, often deeply intertwined with spatial concepts and cultural priorities. These examples provide compelling evidence for linguistic relativity's influence on temporal cognition.
The Aymara's Temporal Orientation: Past Forward, Future Back
The Aymara language, spoken in the Andean highlands, presents a striking contrast to the common "future in front, past behind" metaphor found in English and many other languages. Linguistic analysis reveals a systematic mapping where the past is associated with the space in front of the speaker, and the future with the space behind.
Linguistic Evidence: The core evidence lies in the dual meaning of key spatial terms. The word nayra, meaning "eye," "front," or "sight," is also the primary term used to refer to the PAST. Expressions like nayra mara ("last year," literally "front year") and nayra pacha ("past time," literally "front time") exemplify this usage. Conversely, the word qhipa, meaning "back" or "behind," serves as the basic term for the FUTURE. Examples include qhipüru ("a future day," literally "back day") and qhipa pacha ("future time," literally "back time"). This linguistic pattern is consistent and fundamental to Aymara temporal expression.
Gestural Evidence: Ethnographic studies involving videotaped interviews confirmed that this linguistic pattern extends to non-verbal behavior. Aymara speakers, particularly older individuals less influenced by Spanish, consistently gestured forward, away from their bodies, when discussing past events or times. The extent of the forward gesture often correlated with the temporal distance into the past. In contrast, when referring to the future, speakers gestured backward, often pointing or waving over their shoulder. The present moment (jicha or "now") was typically indicated by gestures pointing downward directly in front of the speaker, signifying co-location. This convergence of linguistic and gestural patterns provides strong evidence for a genuinely different conceptualization of time's spatial orientation.
Conceptual Motivation: Researchers propose that this unique orientation is rooted in the Aymara's epistemic values, particularly the emphasis placed on visual perception as a source of knowledge. The past, having already occurred, is considered "known" or "seen," and thus metaphorically placed in front, within the field of vision. The future, being inherently "unknown" and "unseen," is placed behind the speaker. This suggests that the dominant spatial metaphor for time in Aymara is shaped by a cultural focus on evidentiality and the certainty associated with visual experience.
Mandarin's Vertical Time Axis: Up/Down Metaphors
While English primarily relies on horizontal metaphors (front/back, ahead/behind) to discuss time, Mandarin Chinese frequently and systematically employs vertical spatial metaphors.
Linguistic Evidence: Mandarin speakers use the spatial morphemes shàng ("up" or "above") to refer to earlier points in time and xià ("down" or "below") to refer to later points in time. This vertical mapping applies to the order of events, weeks, months, years, semesters, and more. Common examples include shàng ge yuè (上个月, "last month," literally "up month") and xià ge yuè (下个月, "next month," literally "down month"). While horizontal front/back metaphors (qián 前 "front/before", hòu 后 "back/after") also exist in Mandarin, the use of vertical metaphors is significantly more frequent and systematic than the occasional vertical expressions found in English (e.g., "coming up," "hand down"). One analysis found that a substantial portion (36%) of spatial metaphors for time in Mandarin are vertical. There is also evidence suggesting vertical terms are more common for longer durations (weeks, months), while horizontal terms are more frequent for shorter durations (days, seconds).
Cognitive Evidence: This linguistic pattern correlates with cognitive differences. Experiments have shown that Mandarin speakers demonstrate an implicit cognitive association between time and the vertical axis. For instance, Mandarin speakers were faster to confirm temporal order when stimuli were presented vertically (earlier up, later down) compared to horizontally, while English speakers showed the opposite bias (favoring left-to-right). When asked to physically arrange representations of time, Mandarin speakers were significantly more likely (over 8 times more likely in one study) to create vertical arrangements than English speakers, who overwhelmingly preferred horizontal layouts. Studies involving Mandarin-English bilinguals further support this link, showing that higher proficiency in Mandarin and being tested in a Mandarin context increased the likelihood of using a vertical representation. This suggests that the habitual use of vertical metaphors in the language fosters a corresponding vertical mental timeline.
Potential Origins: The reasons for this vertical orientation are debated, but potential contributing factors include the historical vertical writing direction of Chinese characters.
Kuuk Thaayorre's Landscape Time: Absolute Directions
The Kuuk Thaayorre language, spoken by an Aboriginal community in Pormpuraaw, Australia, offers another fascinating perspective, linking time not to the body's axes (front/back, left/right) but to the absolute coordinates of the landscape.
Spatial Language: Kuuk Thaayorre speakers predominantly use an absolute frame of spatial reference, relying on cardinal directions (north, south, east, west) rather than relative, egocentric terms like "left," "right," "front," or "back". To speak the language correctly, one must maintain constant awareness of their orientation within the cardinal directions. Greetings might involve asking "Which way are you going?", and descriptions commonly refer to directions like "the cup to the north-northeast" or "an ant on your southwest leg". This linguistic requirement cultivates exceptional spatial orientation skills from a young age.
Temporal Representation: This focus on absolute spatial coordinates profoundly influences how Kuuk Thaayorre speakers represent time. When asked to arrange cards depicting temporal progressions (e.g., a man aging, a banana being eaten), they consistently arranged them along an East-to-West axis. This orientation remained constant regardless of the direction the participant was facing during the experiment. If facing south, the sequence went left-to-right; if facing north, it went right-to-left; if facing east, it came towards the body. This demonstrates a non-egocentric representation of time, locked onto the landscape and mirroring the path of the sun, rather than being relative to the observer's body. English speakers, in contrast, consistently arranged the cards from left-to-right, reflecting their writing direction.
Absence of Direct Metaphors: A crucial point is that the Kuuk Thaayorre language itself lacks explicit linguistic metaphors that directly equate time with the East-West axis. They do not commonly speak of time "moving east" or the future being "to the west." Their temporal representation seems to emerge not from direct linguistic mapping of time onto space, but from the deeply ingrained cognitive habit of using an absolute spatial frame of reference, which is constantly reinforced by their spatial language.
These diverse examples powerfully illustrate that human temporal cognition is not monolithic. While mapping time onto space appears to be a common cognitive strategy, the specific spatial dimension and orientation used are highly variable. The Aymara link time to the front/back axis based on visibility and knowledge; Mandarin speakers frequently utilize an up/down vertical axis, possibly influenced by cultural factors like writing systems; and the Kuuk Thaayorre employ an absolute East-West axis tied to their essential navigational framework. These variations correlate strongly with linguistic patterns, but as the Kuuk Thaayorre case demonstrates, the influence may be indirect – stemming from the cognitive habits fostered by the language's structure (particularly its spatial system) rather than solely from direct temporal metaphors. Language, therefore, seems to play a critical role in selecting and reinforcing which spatial schema becomes the dominant framework for conceptualizing and reasoning about time, reflecting different embodied experiences, cultural priorities, or environmental necessities. The deep integration of these temporal frameworks within linguistic structures and their correlation with cognitive patterns suggests a co-evolutionary relationship: languages develop structures to encode culturally salient ways of thinking about time, and these linguistic structures, in turn, shape and stabilize those cognitive patterns for subsequent generations.
The Subjective Clock: Neuroscience of Time Perception
While physics describes time as a dimension within spacetime, and languages offer diverse metaphorical frameworks, neuroscience reveals that our subjective experience of time is an active construction of the brain. There appears to be no single, dedicated "clock" organ measuring objective time. Instead, our sense of duration, passage, and temporal order emerges from the complex interplay of sensory processing, memory, attention, and predictive mechanisms. This constructed nature explains why our internal clock often feels asynchronous with the external world, stretching during moments of fear or boredom and compressing during engaging activities.
Time as a Cognitive Construct
The neuroscientific consensus is shifting away from models based on dedicated pacemakers or accumulators that simply track objective time. Instead, evidence points towards time perception being intrinsically linked to the processing of information content. The brain constructs the experience of time by integrating signals related to changes in the external environment and internal states. Key processes involved include:
Sensory Integration: The continuous flow of information from our senses (sight, sound, touch, etc.) provides the raw material for temporal experience. The rate and complexity of incoming sensory data influence perceived duration.
Memory: Our recollections of past events and their durations provide context and anchors for judging current temporal intervals. Memory contributes to our sense of continuity and personal timeline.
Predictive Processing: Increasingly influential theories propose that the brain operates as a prediction machine, constantly generating hypotheses about the causes of sensory input and updating these predictions based on incoming data. The processing of prediction errors – the mismatch between expectation and reality – is thought to play a crucial role in shaping our perception, including the perception of time.
Perception as Prediction: The "Controlled Hallucination" View
Neuroscientist Anil Seth, building on ideas from others like Chris Frith, articulates a compelling view of perception as a "controlled hallucination". According to this framework, our conscious experience is not a direct readout of external reality, nor is it purely generated internally like a dream or psychotic hallucination. Instead, it is the brain's "best guess" about the state of the world and the body, generated through top-down predictive models. Sensory data acts primarily as error signals, constraining and calibrating these internal predictions. Perception, therefore, is an active process of construction, an "inside-out" generation of experience that is constantly being reined in and updated by reality. This perspective has profound implications for time perception. Our subjective sense of time's flow, its duration, and the ordering of events within it are likely integral components of this ongoing predictive modeling process. The feeling of time passing might be related to the continuous updating of the brain's internal model in response to sensory prediction errors. Deviations from clock time could arise from variations in the rate or intensity of these predictive updates, influenced by factors like attention, emotion, or stimulus complexity. As Seth puts it, "we're all hallucinating all the time. It's just that when we agree about our hallucinations, that's what we call reality". The "control" exerted by sensory input ensures our temporal experience generally aligns with the external world, but the underlying constructive process allows for significant subjectivity.
The Salient Events Model
A concrete neurobiological model linking sensory processing to subjective duration is the "salient events" model. This model proposes that the brain estimates duration by accumulating the number of significant changes, or "salient events," detected during perceptual processing. Salient events are defined operationally as relatively large fluctuations in neural activity within the sensory cortices relevant to the ongoing experience (e.g., visual cortex activity while watching a video). The more salient events registered within a given objective interval, the longer that interval is subjectively perceived to be. This provides a potential neural basis for the common experience that dynamic, changing, or surprising stimuli seem to last longer than monotonous or predictable stimuli of the same physical duration.
Supporting evidence comes from fMRI studies where participants watched silent videos of varying complexity. Researchers developed a computational model that tracked changes in BOLD signals (an indirect measure of neural activity) in the visual cortex. By identifying time points with significant changes (salient events) and accumulating them, the model could successfully predict, on a trial-by-trial basis, whether participants would report a specific video as feeling subjectively longer or shorter compared to others of the same objective length. Notably, the model performed best when using the "signed difference" in neural activity between time points, suggesting the brain might be sensitive not just to the magnitude but also the nature or direction of change, potentially reflecting the processing of prediction errors. Control analyses showed that activity in unrelated sensory areas (auditory, somatosensory cortex) did not predict duration judgments for the visual stimuli, reinforcing the link between modality-specific perceptual processing and subjective time.
The convergence of evidence from predictive processing theories and models like the salient events framework strongly indicates that our perception of time is not mediated by a centralized, abstract clock. Rather, it emerges dynamically from the brain's fundamental task of processing the content of our experiences. The neural mechanisms engaged in understanding what is happening are inextricably linked to constructing our sense of how long it takes. This view implies that subjective time is not a fixed quantity but is inherently plastic and malleable. Given that its construction relies on processes like sensory analysis, prediction, memory, and attention – all of which are subject to learning, adaptation, and influence from factors like emotion and linguistic framing (as discussed in previous sections) – our experience of time can be shaped and potentially altered through various means.
Beyond the Arrow: Exploring Non-Linear Time
The intuitive human experience is dominated by a linear, unidirectional flow of time – the "arrow of time" moving inexorably from a fixed past, through a fleeting present, into an open future. Events occur in sequence, causes precede effects, and entropy generally increases. This "manifest image" of time is deeply embedded in our psychology and often reflected in our language. However, physics and philosophy have long questioned whether this subjective experience accurately reflects the fundamental nature of time. Concepts like eternalism and the block universe challenge the notion of temporal passage, suggesting alternative structures where time might be more akin to a spatial dimension.
Philosophical Frameworks: Eternalism and the Block Universe
Eternalism is the philosophical position holding that all moments in time – past, present, and future – are equally real. This contrasts sharply with presentism (only the present exists) and the growing block theory (past and present exist, but the future does not). Eternalism often draws support from physics, particularly Einstein's theory of relativity, which treats time as a dimension interwoven with space into a four-dimensional spacetime continuum. This leads to the concept of the "block universe". In this view, the entirety of spacetime – all events throughout history from the Big Bang to the distant future – exists as a static, unchanging four-dimensional "block". Time is simply one dimension of this block, analogous to the three spatial dimensions. Just as distant places exist simultaneously, eternalism suggests distant times (past and future events) also "exist" in the same ontological sense. From this perspective, the perceived "flow" or "passage" of time is considered a subjective illusion, an artifact of consciousness moving through or experiencing this static block. As Einstein famously wrote, "People like us, who believe in physics, know that the distinction between past, present, and future is only a stubbornly persistent illusion". Some theological perspectives also align with this, suggesting God exists outside of time and perceives the universe as a complete block. The block universe model, while finding resonance with relativity, faces significant challenges and criticisms. Many physicists and philosophers argue it provides a deeply inadequate account of our experience of change, agency, and the apparent openness of the future. It raises difficult questions about determinism and free will: if the future already exists within the block, are our choices predetermined? Some argue that quantum mechanics, particularly phenomena like quantum measurement or black hole evaporation, might challenge the static block view and support an objective passage of time. Physicist Lee Smolin, for instance, advocates for the reality of time's passage and proposes alternative models like a "thick present" where causal processes generate future events from present ones. The debate reflects a fundamental tension between the timeless, symmetric descriptions often favored by fundamental physics and the asymmetric, dynamic nature of lived experience.
Cognitive Science and Non-Linearity
The neuroscientific view of time as a cognitive construct adds another layer to this discussion. If our experience of time is generated by the brain, it raises the possibility that the brain could, in principle, construct or represent temporal experiences that deviate from strict linearity, irrespective of the underlying physical structure of time. Some speculative work explores this very idea. Could consciousness itself be distributed non-linearly across time? This hypothesis suggests awareness might not be confined to the immediate present but could potentially access or interact with past or future states in ways beyond conventional memory recall or probabilistic prediction. Such a concept challenges standard notions of causality and agency, implying a form of direct interaction with future events rather than inference based on past patterns. While highly speculative, this line of thought pushes the boundaries of how we conceive the relationship between mind and time. A less radical thought experiment highlights the potential divergence between physical and cognitive time. Imagine two individuals counting mentally at their natural pace, one on Earth and one near a massive object experiencing significant gravitational time dilation. According to relativity, physical time passes slower for the individual in the strong gravitational field. However, the hypothesis suggests that their subjective experience of counting – the perceived interval between saying "one" and "two" – would remain unchanged from their own perspective.
This implies the existence of a "cognitive time" constructed internally, which can remain stable even when external "physical time" is distorted, further supporting the idea that our temporal experience is not simply a reflection of external physics.
Fictional narratives often explore these themes, using non-linear timelines (as in films like "Arrival" or novels like "Slaughterhouse-Five") as thought experiments to examine how different temporal structures might impact perception, causality, memory, and free will.
While the block universe remains a contentious model for describing physical reality or human consciousness, its core idea – the co-reality and potential accessibility of different time points – offers an intriguing, albeit metaphorical, framework for considering how advanced AI might represent and interact with time. An AI system, particularly one operating in a deterministic or highly predictable domain, could potentially treat historical, current, and predicted future data as part of a single, accessible continuum for analysis. AI's capacity for massive parallel processing and pattern recognition across vast datasets aligns conceptually with the idea of accessing and relating information across different temporal points simultaneously, moving beyond the constraints of linear, sequential human experience. This is particularly relevant for AI systems designed for prediction, where analyzing past patterns to forecast future events essentially treats the future as an accessible continuation of the past, echoing the block universe's timeless perspective. Ultimately, the philosophical and physical debates surrounding non-linear time underscore a significant disconnect between theoretical models (often timeless and symmetric) and the fundamental human experience of unidirectional temporal flow. This presents a critical choice for AI development:
Should AI aim to model the subjective, potentially illusory, human experience of time's passage, or should it strive to implement a more abstract, potentially non-linear model derived from physics or computational principles? The path chosen will profoundly shape the capabilities, limitations, and interactive nature of future intelligent systems.
Artificial Timekeepers: AI's Representation of Time
Having explored the human dimensions of time perception shaped by language, cognition, and potentially non-linear concepts, the focus now shifts to how Artificial Intelligence currently models and processes temporal information. The dominant paradigms, particularly Recurrent Neural Networks (RNNs) and Transformer models, have achieved significant success in handling sequential data, where order is paramount. However, their underlying mechanisms reveal specific, often implicit, ways of representing time, primarily rooted in linear progression.
Recurrent Neural Networks (RNNs): Sequential Processing and Memory
RNNs were among the first neural network architectures specifically designed to handle sequential data like text, speech, or time series. Their core innovation lies in their recurrent connections: the output from processing one element in a sequence is fed back as input when processing the next element.
Architecture and Mechanism: An RNN processes an input sequence (xt) one step at a time. At each time step t, the network takes the current input xt and combines it with its hidden state (st−1) from the previous step. This hidden state acts as a form of memory, accumulating information about the preceding elements in the sequence. The network applies learned transformations (using weight matrices U, W, V) to the current input and previous hidden state to compute the new hidden state (st) and potentially an output (ot) for that time step. Crucially, the same set of weights (parameters) is used at every time step, allowing the model to handle sequences of varying lengths.
Time Representation: In RNNs, time is represented implicitly through the sequential nature of the computation and the evolution of the hidden state. The network learns temporal dependencies by virtue of how its internal memory changes as it steps through the sequence. The order of processing directly encodes the temporal order of the data.
Limitations: While effective for capturing short-term dependencies, standard RNNs struggle with long sequences due to the vanishing and exploding gradient problem. During training (using Backpropagation Through Time, BPTT), gradients propagated back through many time steps can become infinitesimally small (vanish) or excessively large (explode), hindering the model's ability to learn relationships between distant elements in the sequence. Variants like Long Short-Term Memory (LSTM) and Gated Recurrent Units (GRU) were developed to address this by introducing gating mechanisms that allow the network to selectively retain or forget information over longer periods. However, even these advanced RNNs remain fundamentally sequential, meaning computation at step t depends on completing step t-1. This inherent sequentiality limits parallelization during training and inference, making them slow for very long sequences or large datasets.
Transformer Models: Attention, Parallelism, and Positional Encoding
Introduced in the landmark 2017 paper "Attention Is All You Need," Transformer models revolutionized sequence processing, particularly in Natural Language Processing (NLP), by dispensing with recurrence altogether.
Architecture and Mechanism: Transformers rely primarily on a mechanism called self-attention. Instead of processing tokens one by one, the self-attention mechanism allows the model to weigh the importance of all tokens in the input sequence simultaneously when computing the representation for each individual token. It calculates "attention scores" between pairs of tokens, indicating how relevant one token is to another within the context of the entire sequence. These scores are then used to create context-aware embeddings for each token. Transformers typically employ multi-head attention, allowing the model to attend to different types of relationships in parallel. The overall architecture often consists of an encoder (to process the input sequence) and a decoder (to generate an output sequence), each built from stacked layers of self-attention and standard feed-forward networks.
Handling Sequences without Recurrence: The key advantage of the attention mechanism is that it enables parallel processing of the entire sequence. Since the computation for each token can consider all other tokens directly, there is no need to wait for previous steps to complete.
Positional Encoding: Because the self-attention mechanism itself is permutation-invariant (it doesn't inherently know the order of tokens), Transformers require an explicit way to incorporate sequence order information. This is achieved through positional encoding. Vectors representing the absolute or relative position of each token are added to the initial token embeddings before they enter the attention layers. These encodings provide the model with the necessary information about the sequence structure.
Time Representation: In Transformers, time (or sequence order) is represented explicitly through positional encodings and contextually through the self-attention mechanism. Positional encodings provide the absolute or relative location, while attention allows the model to directly model dependencies between tokens regardless of their distance in the sequence (within the model's context window).
Advantages: Transformers generally outperform RNNs in capturing long-range dependencies, as attention provides a more direct path for information flow between distant elements. Their high parallelizability allows for significantly faster training on large datasets using modern hardware like GPUs and TPUs.
Limitations: The computational complexity of the standard self-attention mechanism is quadratic with respect to the sequence length (O(N2)), making it expensive for very long sequences. Furthermore, Transformers operate within a fixed context window, meaning they cannot model dependencies between tokens that fall outside this predefined length limit.
Comparative Table: RNNs vs. Transformers in Temporal Processing
The following table summarizes the key differences between RNNs and Transformers in how they handle sequential data and represent time:
Despite their architectural differences, both RNNs and Transformers fundamentally operate on the principle of sequential order. RNNs achieve this through their step-by-step updates, inherently linking the processing of an element to its predecessor. Transformers, while processing in parallel, explicitly inject sequence order using positional encodings, ensuring the model is aware of the linear arrangement of the input.
This shared reliance on sequence position reflects a bias towards the linear progression of time as commonly experienced, but it may also constrain these models' ability to represent or reason about more complex, non-sequential, or multi-faceted temporal phenomena found in the real world or suggested by human cognitive diversity.
Speculating Beyond Sequences: AI and Non-Linear Time
The successes of RNNs and Transformers notwithstanding, their inherent focus on linear sequences limits their ability to capture the full complexity of temporal dynamics in the real world. Phenomena involving intricate causal chains unfolding over long durations, simultaneous events with complex interdependencies, cyclical patterns, or the nuanced temporal references found in human language often demand more sophisticated representational frameworks. Recognizing these limitations, AI research is actively exploring alternative approaches and theoretical concepts that might enable machines to represent and reason about time in richer, potentially non-linear ways.
Theoretical AI Research & Concepts Moving Beyond Linearity
Several research avenues suggest a move towards more diverse temporal modeling in AI:
Foundation Models for Time Series: A significant trend is the development of large, pre-trained "foundation models" specifically for time series analysis. Models like TimeGPT-1, Lag-Llama, Chronos, TimesFM, MOIRAI, and Tiny Time Mixers (TTM) aim to generalize across diverse time series datasets, often enabling "zero-shot" forecasting (predicting on unseen data without task-specific retraining). These models leverage large-scale pre-training and architectures sometimes diverging from standard Transformers (e.g., Chronos uses tokenization and T5, while TTM uses MLP-based mixers instead of attention). This signifies a shift towards models that learn more general temporal patterns rather than just sequence-specific dependencies.
Integrating Non-Sequential Context: Researchers are exploring ways to augment sequential models like Transformers with non-sequential information. This might involve encoding static attributes, user profiles, or graph structures as context vectors and incorporating them into the model, for instance, by concatenating them to input embeddings or using them to condition attention mechanisms. This allows models to consider fixed contextual factors alongside the evolving sequence. Graph-based approaches, like GraphMaker for generating attributed graphs or Graph Neural Networks for time series, explicitly model relational structures that may not be purely sequential.
Alternative Architectures and Programming Models: Beyond Transformers and RNNs, other architectures are being investigated. Structured State Space sequence models (S4) offer a different mathematical framework aimed at efficiently modeling very long sequences, potentially overcoming some limitations of Transformers. More radical proposals include step-variant, non-sequential programming models where execution flow is driven dynamically by the state of the data itself, rather than a predetermined sequence of instructions, allowing for parallel, data-driven logic formation.
Temporal Reasoning Enhancements: Recognizing the difficulty models face in reasoning across time, benchmarks like REXTIME are being created to specifically evaluate the ability to connect information from different segments of a video to answer causal or relational questions. The limited performance of even large models on such tasks highlights the need for improvement. Techniques like TIMER-Instruct focus on instruction tuning that explicitly considers temporal dependencies within longitudinal data (e.g., clinical records) to enhance temporal reasoning capabilities.
Temporal Logic Integration: There is growing interest in combining the formal power of temporal logic with AI models. Temporal logics like Linear Temporal Logic (LTL), Probabilistic Computation Tree Logic (PCTL), or specialized logics like CPLTL provide precise languages for expressing complex temporal properties (e.g., "event A always precedes event B," "event C will eventually happen," "if event D occurs, event E will hold until F occurs"). Integrating these logics with LLMs or reinforcement learning agents could enable more sophisticated planning, verification, and reasoning about dynamic processes over time. Research explores using PCTL formulas derived from time-course data to represent and infer causal relationships, including time delays and probabilities.
Causal Inference in Temporal Settings: Perhaps the most critical direction is the integration of causal inference principles into temporal AI models. Standard sequence models excel at identifying correlations but often fail to distinguish them from true causation. Understanding causality is vital for robust prediction, effective planning, and reliable decision-making, especially in high-stakes domains like healthcare or autonomous driving. Frameworks like Structural Equation Models (SEMs), Temporal-Logic-based Causal Diagrams (TL-CDs), or Causal Logic Models (CLMs) are being adapted or developed to represent causal relationships that unfold over time. These models allow reasoning about the effects of interventions (manipulating variables) and counterfactuals ("what would have happened if...") in a temporal context. Statistical methods like Granger causality provide tools for testing predictive causality in time series data, though with specific assumptions. Combining causal graphs with temporal logic enables reasoning about the temporal dynamics of causal mechanisms.
These diverse research threads suggest a potential future where AI moves beyond simple linear sequence processing. The emphasis on causality and temporal logic points towards AI systems that don't just predict the next event based on past patterns, but understand the underlying causal mechanisms that generate those patterns over time. This deeper understanding is crucial. For AI to progress from pattern matching to genuine comprehension of dynamic systems, it must incorporate a notion of causality that operates within a temporal framework. Integrating formalisms from causal inference and temporal logic provides a pathway towards AI that can reason about why things happen in sequence, predict the consequences of actions more reliably, and potentially plan more effectively in complex, evolving environments. Furthermore, the emergence of foundation models for time series, specialized temporal reasoning benchmarks, and alternative architectures signals a diversification in how AI approaches time.
Instead of relying solely on general-purpose sequence processors like Transformers, the field is developing more specialized tools tailored to different facets of temporal data – forecasting, anomaly detection, causal analysis, cross-event reasoning. This trend suggests a move away from a monolithic view of temporal processing towards a richer, more varied toolkit, potentially enabling AI to handle the multifaceted nature of time in a more flexible and powerful manner, perhaps eventually mirroring some of the cognitive diversity observed in humans.
Implications for Intelligent Machines
The prospect of AI systems capable of representing and reasoning about time in ways that transcend simple linear sequences holds profound implications, offering significant potential advantages while also presenting substantial challenges. Moving towards richer temporal models, incorporating non-linearity, causality, and logical structure, could fundamentally alter AI capabilities across numerous domains.
Advantages and Challenges of Non-Linear/Richer Temporal AI
Complex Planning and Prediction: AI equipped with a deeper understanding of temporal dynamics, including non-linear relationships and causal mechanisms, could excel at long-term planning and prediction in complex, dynamic environments. Instead of merely extrapolating trends from sequential data, such systems could model underlying causal processes, anticipate delayed consequences, evaluate multiple potential futures simultaneously, and devise more robust strategies. This capability would be invaluable in fields like economics, climate modeling, logistics, and strategic game playing.
Enhanced Causal Understanding: A primary benefit lies in the ability to perform robust causal inference on temporal data. This allows AI to move beyond identifying correlations (e.g., event A often precedes event B) to understanding causation (e.g., event A causes event B after a specific delay under certain conditions). Disentangling correlation from causation is critical for reliable decision-making. AI capable of understanding temporal causality could better predict the effects of interventions over time, assess risks, diagnose problems, and provide trustworthy explanations for its reasoning. This is crucial for applications in medicine (treatment effects), finance (market dynamics), autonomous systems (safety and reliability), and policy-making.
Improved Human-AI Interaction: Humans naturally use complex and varied temporal language, employing different metaphors and referencing subjective experiences of time. AI systems that can understand and perhaps even model these nuances – recognizing the difference between Aymara's past-in-front and English's future-in-front, or grasping the implications of vertical time metaphors in Mandarin – could interact with humans more naturally, effectively, and empathetically. AI capable of understanding subjective time could also be valuable in applications related to user experience design or mental health monitoring.
Accelerated Scientific Discovery: Many scientific domains grapple with complex systems evolving over time, from gene regulatory networks and neural activity patterns to ecological dynamics and cosmological evolution. AI with advanced temporal reasoning capabilities, particularly those integrating causality and logic, could serve as powerful tools for analyzing vast datasets, uncovering hidden temporal patterns, identifying causal relationships, testing hypotheses, and ultimately accelerating scientific progress.
Despite the potential benefits, developing AI with sophisticated non-linear or causal temporal reasoning faces significant hurdles:
Computational Complexity: Models that incorporate complex logical structures, detailed causal graphs, or non-sequential access mechanisms are likely to be significantly more computationally expensive than standard sequential models like Transformers. Training these models may require vast computational resources and time, potentially limiting their practical deployment. The quadratic complexity of self-attention in Transformers already poses challenges for very long sequences; more complex temporal models could exacerbate these issues.
Theoretical Foundations: Establishing robust theoretical frameworks for non-linear time representation and reasoning in AI is a major challenge. How should concepts like branching time, cyclical time, or the block universe be formally represented in a way that is computationally tractable and semantically meaningful for AI? Ensuring logical consistency and defining appropriate learning algorithms for such representations remain open research questions.
Data Requirements: Learning complex temporal dynamics and causal relationships likely requires massive and diverse datasets that capture these phenomena accurately. Acquiring such data, especially data suitable for causal inference (e.g., including interventions or counterfactuals), can be difficult and expensive.
Interpretability and Trust: As AI models become more complex in their temporal reasoning, understanding how they arrive at their conclusions becomes increasingly difficult. Explaining the reasoning of an AI operating with non-linear time or intricate causal logic poses a significant challenge for interpretability and building trust, particularly in high-stakes applications.
Grounding and Alignment: Ensuring that abstract or non-linear temporal models remain grounded in and useful for interacting with the physical world is crucial. There is a risk of developing models that are theoretically interesting but fail to align with the temporal realities relevant to specific tasks or human interaction. How does an AI operating with a "block universe" representation effectively interact with a user experiencing linear time?
The pursuit of AI with richer temporal capabilities inevitably involves navigating a fundamental trade-off between representational power and practical feasibility. While incorporating non-linear structures, causal inference, and temporal logic offers the potential for deeper understanding and enhanced performance on complex temporal tasks, these sophisticated representations introduce significant challenges. They increase model complexity, demanding greater computational resources for training and inference, requiring new theoretical developments, and making the models potentially harder to interpret and trust. In contrast, simpler sequential models like RNNs and Transformers, while limited in their temporal expressiveness, are comparatively better understood and computationally more manageable for many current tasks. Therefore, advancing temporal AI requires careful consideration of this trade-off, balancing the ambition for more human-like temporal intelligence with the constraints imposed by computation, data availability, theoretical understanding, and the need for interpretable, reliable systems.
Synthesis: Human Time Cognition as a Blueprint for AI?
The journey through linguistic relativity, cross-cultural temporal concepts, neuroscientific construction of time, philosophical debates, and AI's current and potential temporal modeling reveals a complex and fascinating landscape. Human temporal cognition emerges not as a monolithic reflection of physical time, but as a flexible, dynamic, and culturally modulated construct, deeply intertwined with language and predictive processing. This stands in contrast to the largely linear, sequence-bound representations currently dominant in AI. Synthesizing these diverse threads offers valuable perspectives on how the richness of human time experience might inform the future development of artificial intelligence. The evidence reviewed consistently points to the profound influence of language on how humans structure and conceptualize time. From the front-facing past of the Aymara to the vertical timeline of Mandarin speakers and the landscape-anchored time of the Kuuk Thaayorre, linguistic frameworks provide cognitive scaffolding that shapes habitual ways of thinking about temporal relationships. Neuroscience complements this picture by demonstrating that the subjective experience of time is actively constructed by the brain, emerging from the interplay of sensory processing, memory, and predictive mechanisms, rather than being passively measured.
Our internal clock is intrinsically linked to the content of our experience and operates as a form of "controlled hallucination," the brain's best guess about the unfolding world. Philosophical inquiries further challenge the intuitive linearity of time, proposing models like the block universe where past, present, and future coexist.
Current AI, primarily through RNNs and Transformers, excels at processing sequential data but largely operates within a linear framework, implicitly representing time through order and state changes or explicitly via positional encodings. While powerful, this approach may not capture the full spectrum of temporal reasoning required for deeper understanding and interaction with the world. Emerging research directions, however, signal a move towards richer temporal models incorporating causality, temporal logic, foundation models for time series, and non-sequential context integration. The remarkable flexibility of human temporal cognition serves as a powerful source of inspiration for AI. Humans effortlessly switch between different temporal frames – thinking linearly about schedules, cyclically about seasons, using diverse spatial metaphors, reasoning about cause and effect across time, imagining possible futures, and experiencing subjective variations in duration. This adaptability, shaped by language and culture, highlights a key difference from the relative rigidity of current AI. The diversity observed across languages like Aymara, Mandarin, and Kuuk Thaayorre is not merely an anthropological curiosity; it demonstrates the adaptive power of different cognitive strategies for organizing time, each potentially suited to specific contexts or cultural needs. This human cognitive diversity should be viewed as a feature, not a bug, offering a rich portfolio of potential mechanisms and representations for AI.
This suggests several pathways for informing AI design:
Moving Beyond Monolithic Models: Instead of seeking a single, universal temporal representation for AI, perhaps future systems could benefit from incorporating multiple, context-dependent frameworks, drawing inspiration from human linguistic and cognitive flexibility. An AI might learn to employ linear models for scheduling tasks but switch to cyclical models for seasonal pattern analysis or causal models for diagnostic reasoning.
Integrating Causality and Logic: The synthesis strongly reinforces the need for AI to move beyond correlational sequence modeling towards incorporating causal inference and potentially temporal logic. This is essential for achieving robust prediction, planning, and explanation in dynamic environments. Human reasoning fluidly integrates sequence, duration, and causality; AI must strive for similar integration.
Modeling Subjectivity: While challenging, exploring AI models that can understand or even predict human subjective time perception could open new avenues for human-computer interaction, personalized assistants, adaptive learning systems, and applications in mental health or well-being.
Learning Diverse Temporal Concepts: A key challenge is developing AI systems that can learn diverse temporal concepts and representations from data, including language and interaction, much like humans do. This might involve meta-learning approaches or architectures designed for greater representational flexibility.
The exploration of time across disciplines reveals that achieving human-like temporal understanding in AI is not merely about processing sequences faster or with longer memory. It requires grappling with the constructed nature of time, the influence of representational frameworks (like language), the importance of causality, and the inherent flexibility that characterizes human cognition. Human temporal diversity provides a valuable blueprint, suggesting that the path towards more temporally intelligent AI may lie in embracing multiple representational strategies and integrating causal reasoning, rather than perfecting a single, linear, sequential paradigm.
Time, Thought, and the Future of Artificial Intelligence
The relationship between language, the cognitive construction of time, and the potential for Artificial Intelligence is intricate and deeply interconnected. This article has traversed diverse fields – linguistics, neuroscience, philosophy, and computer science – to illuminate how time, far from being a simple objective measure, is profoundly shaped by the human mind and the languages we use. Linguistic relativity suggests that the structures of our native tongues provide cognitive frameworks that influence how we segment, spatialize, and reason about temporal events, as vividly illustrated by the contrasting temporal worlds of Aymara, Mandarin, and Kuuk Thaayorre speakers. Neuroscience further reveals time as an active construction of the brain, emerging from predictive processing and the integration of sensory information, resulting in a subjective experience that often diverges from the steady tick of the clock. Philosophical inquiries push these boundaries further, questioning the very linearity and passage of time that seems so intuitive. Current AI, embodied primarily in sequential architectures like RNNs and Transformers, has made remarkable strides in processing ordered data but largely reflects a linear conception of time, encoded implicitly through state transitions or explicitly through positional information. While effective for many tasks, this approach falls short of capturing the richness, flexibility, and causal depth of human temporal cognition.
The future trajectory of AI's engagement with time points towards a necessary evolution beyond simple sequence modeling. Research into foundation models for time series, the integration of temporal logic and causal inference, and the development of benchmarks for cross-time reasoning signals a growing recognition that deeper temporal understanding is crucial for the next generation of intelligent systems. The ability to grasp causality unfolding over time, to reason about complex temporal relationships formally, and potentially to represent time in more flexible, non-linear ways holds the key to unlocking AI capabilities in complex planning, reliable decision-making, nuanced human interaction, and scientific discovery.
Ultimately, the human experience of time – subjective, constructed, linguistically mediated, and remarkably flexible – serves not merely as a point of contrast, but as a source of inspiration for AI.
The cognitive diversity demonstrated across cultures provides a blueprint for designing AI systems that are more adaptive, context-aware, and capable of handling the multifaceted nature of time in the real world. Understanding the intricate tapestry woven from time, thought, and language is therefore fundamental, not only for comprehending the human condition but also for charting the course towards artificial intelligence that can truly navigate, reason about, and interact meaningfully within our dynamic world.
Comments