As the artificial intelligence industry continues to expand rapidly, investors are increasingly turning their attention to the financial performance of AI-focused companies. One area that stands out is the cost of goods sold (COGS) - a metric that often differs significantly between AI companies and their counterparts in the traditional tech sector.
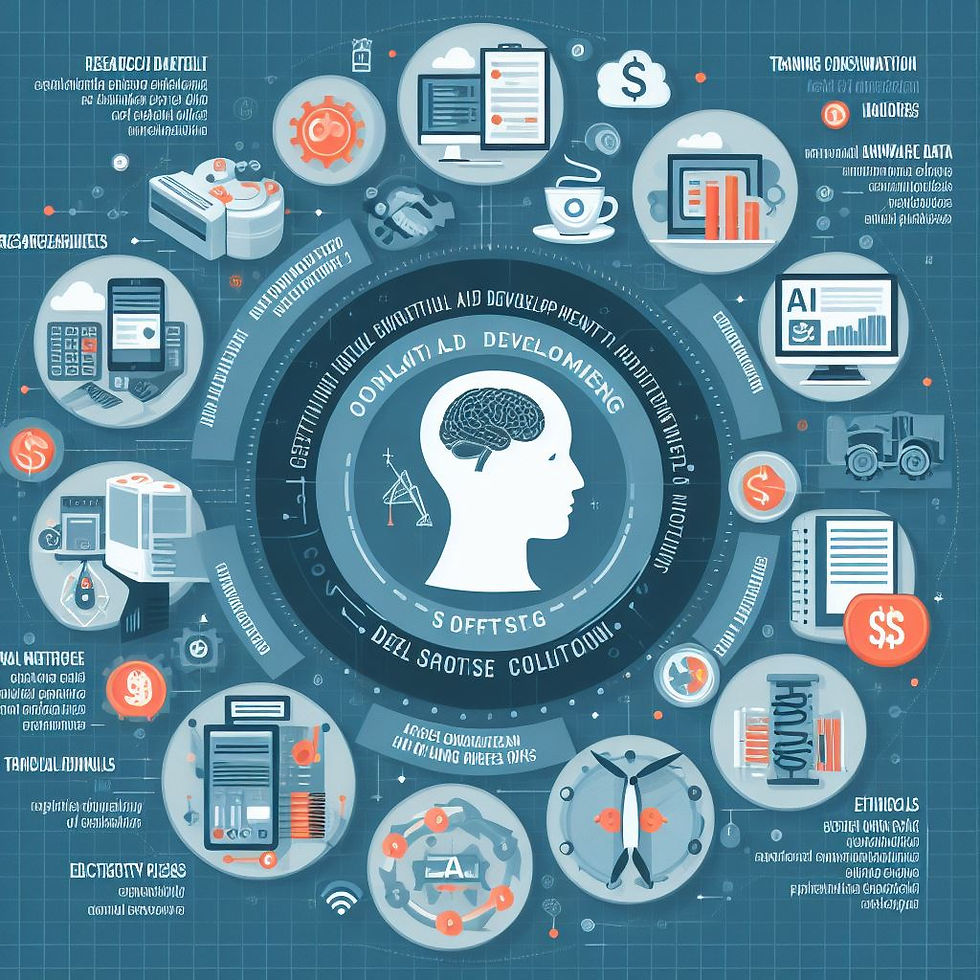
The Traditional Tech COGS Model
In traditional software and tech companies, COGS primarily consists of the direct costs associated with delivering a product or service to customers. The key characteristic is that these costs are generally relatively low compared to the overall revenues, allowing tech firms to achieve attractive gross margins.
The Unique Challenges of AI COGS
AI companies, on the other hand, face a distinctly different COGS landscape. Here's why:
Data Acquisition and Curation: Building high-performance AI models requires vast amounts of high-quality training data. Acquiring, cleaning, and curating this data can be an immensely resource-intensive process, often accounting for a significant portion of an AI company's COGS. Example: A Large Language Model (LLM) focused company may need to scour the internet, purchase datasets, and employ teams of data annotators to label millions of text samples before they can train their language models. These data-related costs can easily eclipse the costs of hosting the actual AI models in the cloud.
Compute Power Requirements: Training and running sophisticated AI models requires massive amounts of computational power, often utilizing GPU-accelerated infrastructure. The energy and infrastructure costs associated with these compute-heavy workloads can be staggering. Example: Some AI research labs are spending hundreds of millions on GPU hardware and electricity bills in a single year to power their large language model experiments.
Model Development and Iteration: Developing and iterating on AI models is a highly iterative and experimental process. It often involves running numerous experiments, testing different architectures and hyperparameters, and fine-tuning models - all of which contribute to the COGS. Example: A computer vision startup may need to train dozens of image recognition models, each requiring hundreds of GPU-hours, before settling on the optimal architecture for their product.
Talent Acquisition and Retention: Building and operating AI systems requires highly specialized technical talent, from machine learning engineers to data scientists. Attracting and retaining this top-tier talent comes at a premium, adding to the COGS. Example: Leading AI research labs and tech companies often offer multimillion-dollar compensation packages to lure the best AI researchers and engineers away from academia and competitors.
The Implications for Investors
These unique COGS dynamics have several implications for investors evaluating AI companies:
Gross Margins May Be Lower: Due to the high data, compute, and talent costs, AI companies often have lower gross margins compared to traditional software or tech firms. Investors should adjust their expectations accordingly.
Scale and Efficiency are Key: AI companies that can achieve significant scale and operational efficiency will be better positioned to improve their COGS over time and drive higher profitability.
Long-Term Investment Horizon: Investing in AI companies often requires a longer-term outlook, as they may need to prioritize model development and infrastructure investment over short-term profitability.
Strategies for Managing AI COGS
While the elevated COGS in AI companies may pose a challenge, there are several strategies these firms can employ to optimize their cost structure and drive long-term profitability:
Data Efficiency and Automation: AI companies can invest in techniques like active learning, data augmentation, and synthetic data generation to reduce their reliance on manual data curation and labeling. Automating these processes can significantly lower data-related COGS. Example: A computer vision startup leveraging techniques like generative adversarial networks (GANs) to synthetically expand their training dataset, reducing the need for expensive manual annotation.
Technological Advancements in Hardware: As the AI hardware ecosystem evolves, companies can take advantage of increasingly powerful and energy-efficient chips and infrastructure. This can help lower the compute-related COGS over time. Example: A leading AI research lab migrating their models to run on the latest generation of custom-designed AI accelerators, which offer significantly improved performance-per-watt.
Operational Efficiency and Optimization: AI companies can streamline their model development and experimentation processes, leveraging techniques like automated hyperparameter tuning and model architecture search. This can help reduce the costs associated with model iteration.
Strategic Partnerships and Outsourcing: AI companies can explore partnerships with cloud providers, data vendors, and specialized service providers to gain access to resources and expertise at a lower cost. Selectively outsourcing certain functions can help manage COGS.
By implementing these strategies, AI companies can work to optimize their COGS over time, positioning themselves for sustained profitability and growth in the rapidly evolving AI landscape.
As the AI industry continues to evolve, understanding the unique COGS challenges faced by these companies will be crucial for investors to make informed decisions and identify the most promising opportunities.
Comentarios