The Anthropogenic Debt Deepens: Training Data, Copyrights, and the Inheritance of Bias
- Aki Kakko
- Mar 27
- 5 min read
Continuing our exploration of anthropogenic debt in AI, we must delve deeper into the thorny issues surrounding training data, copyrights, and the pervasive inheritance of biases. These elements are inextricably linked to the human effort that fuels AI models and significantly shape their capabilities and limitations. Understanding these complexities is crucial for responsible AI development and deployment.
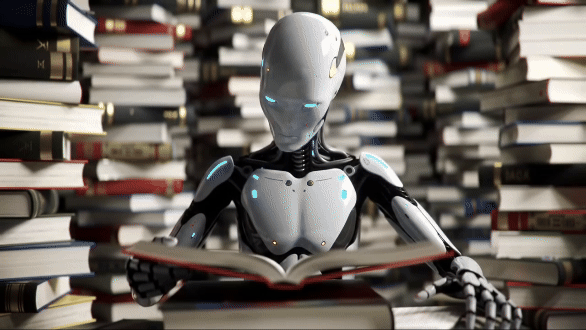
The Training Data Labyrinth: A Tangled Web of Rights and Realities
The lifeblood of any AI model is its training data: the vast datasets used to teach the model to recognize patterns, make predictions, and generate outputs. These datasets are rarely pristine and often represent a complex amalgamation of publicly available information, copyrighted materials, and data collected from individuals with varying degrees of consent.
Copyright Implications:
Many large language models and image generation models are trained on datasets that include copyrighted materials like books, articles, images, and music. While the use of copyrighted material for training AI models falls under the umbrella of "fair use" in some jurisdictions, the legal boundaries remain blurry. The core argument rests on whether the AI model's output is considered a derivative work of the copyrighted material used in training. This ambiguity has led to lawsuits and debates over intellectual property rights and the ownership of AI-generated content. For example, if an AI model is trained on the works of a particular author and subsequently generates text that closely resembles that author's style, does the author have a claim of copyright infringement? The answer is complex and often depends on the specific circumstances. This raises fundamental questions about the balance between fostering innovation and protecting the rights of creators.
Data Sourcing and Consent:
The provenance of training data is another critical concern. How was the data collected? Did individuals whose data was used provide informed consent? Are there ethical considerations surrounding the use of data from vulnerable populations or sensitive domains? The answers to these questions are not always readily available, making it difficult to assess the ethical implications of using specific datasets. Scraping data from the internet, even publicly available data, without considering the terms of service or the expectations of users can raise serious ethical concerns. Furthermore, the aggregation of data from multiple sources can create new privacy risks and exacerbate existing biases.
The "Ghost Work" of Data Labeling:
A significant portion of training data requires human annotation and labeling. This often involves low-paid workers performing repetitive and tedious tasks, such as labeling images, transcribing audio, and categorizing text. This "ghost work" is essential for training AI models but is often overlooked and undervalued. The working conditions of these data labelers can be precarious, and their contributions are often not adequately recognized. Furthermore, the biases and perspectives of these labelers can inadvertently influence the model's behavior.
The Inheritance of Bias: From Data to Algorithms
Perhaps the most insidious consequence of anthropogenic debt is the inheritance and amplification of biases embedded within training data. AI models are not inherently neutral; they learn from the data they are trained on, and if that data reflects societal biases, the model will inevitably perpetuate and potentially exacerbate those biases.
Gender Bias: Language models trained on datasets dominated by male-authored texts can exhibit gender biases in their outputs. For example, they might associate certain professions (e.g., doctor, CEO) with male pronouns more frequently than female pronouns. Similarly, image generation models trained on biased datasets can perpetuate harmful stereotypes about gender roles and appearances.
Racial Bias: Facial recognition systems trained primarily on images of white faces have been shown to be less accurate at identifying individuals with darker skin tones. This can lead to discriminatory outcomes in law enforcement and other applications. Language models can also exhibit racial biases by associating certain ethnicities with negative stereotypes or using biased language when describing individuals from those groups.
Socioeconomic Bias: Data used to train AI models for loan applications, hiring decisions, or criminal justice can reflect existing socioeconomic disparities. This can lead to discriminatory outcomes that perpetuate cycles of poverty and inequality.
Examples of Bias in AI:
AI Recruiting Tool: Well known major corporation developed an AI-powered recruiting tool to screen job applicants. However, the tool was found to be biased against women because it was trained on a dataset of resumes that were predominantly submitted by men. The tool penalized resumes that contained words associated with women's colleges or women's organizations.
COMPAS Recidivism Algorithm: The COMPAS algorithm is used by courts in the United States to assess the risk of recidivism among criminal defendants. However, ProPublica found that the algorithm was biased against black defendants, falsely labeling them as higher risk more often than white defendants.
Google's Image Recognition: Google's image recognition system has been known to misidentify images of black people, sometimes labeling them as gorillas or other primates.
Addressing Bias: A Multifaceted Approach
Mitigating bias in AI requires a multifaceted approach that addresses the problem at multiple levels:
Data Auditing and Curation: Carefully audit training data to identify and mitigate sources of bias. Prioritize the collection of diverse and representative datasets that accurately reflect the population.
Algorithmic Fairness Techniques: Develop and implement algorithms that are designed to be fair and equitable. This might involve using techniques such as adversarial debiasing, which aims to remove biases from the model's representations.
Transparency and Explainability: Ensure that AI systems are transparent and explainable, allowing humans to understand how they arrive at their decisions. This can help to identify and correct biases that might be hidden within the model.
Human Oversight and Accountability: Maintain human oversight of AI systems to ensure that they are not used in discriminatory or harmful ways. Establish clear lines of accountability for AI-related decisions.
Ethical Guidelines and Regulations: Develop ethical guidelines and regulations for the development and deployment of AI systems to prevent bias and promote fairness.
Recognizing the Full Extent of the Debt
The anthropogenic debt of AI extends beyond the initial human effort required to build and train models. It encompasses the complex issues surrounding training data, copyrights, and the inheritance of bias. Ignoring these factors can lead to a distorted view of AI's capabilities and potentially harmful consequences. By acknowledging the full extent of this debt, we can work towards developing AI systems that are more responsible, equitable, and beneficial to all of humanity. This requires a collaborative effort involving researchers, developers, policymakers, and the public to ensure that AI is used to promote fairness, justice, and opportunity for everyone. Only through a conscious and concerted effort can we hope to repay the anthropogenic debt and build a future where AI serves as a force for good.
Comments