Artificial Intelligence is rapidly evolving, permeating various aspects of our lives, from healthcare and finance to entertainment and transportation. However, beneath the surface of sophisticated algorithms and vast datasets lies a subtle but pervasive problem: anthropocentric bias. This is the tendency to view the world solely from a human perspective, projecting human assumptions, values, and limitations onto non-human entities, including AI. This article delves into the intricacies of anthropocentric bias in AI, exploring its origins, manifestations, consequences, and potential solutions.
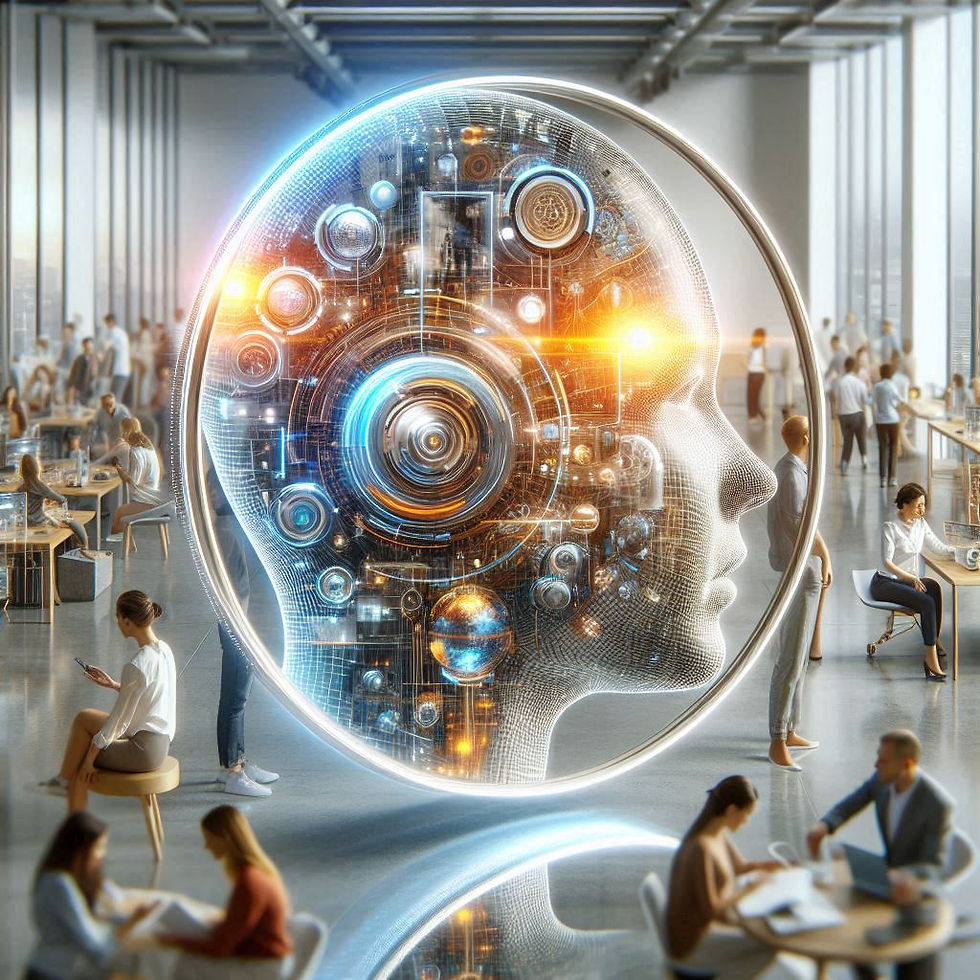
What is Anthropocentric Bias?
At its core, anthropocentric bias is the belief that humanity is the central or most significant entity in the universe. It manifests in our tendency to:
Assume human-like intelligence: Believing that AI needs to think, feel, and reason like humans to be considered intelligent.
Prioritize human needs and values: Designing AI systems that primarily cater to human desires and preferences, often at the expense of other considerations.
Judge AI based on human standards: Evaluating AI performance based on how well it imitates human capabilities, rather than on its inherent abilities or potential benefits.
The Roots of Anthropocentric Bias in AI Development
Anthropocentric bias in AI stems from several contributing factors:
Human Developers: AI systems are built, trained, and evaluated by humans. Our inherent biases, whether conscious or unconscious, inevitably influence the design and functionality of these systems.
Human Data: AI algorithms learn from data, and much of that data is generated by humans and reflects human perspectives, societal norms, and historical inequalities.
Human Interaction: AI is often designed to interact with humans, leading to an emphasis on human-interpretable explanations, human-compatible communication styles, and human-pleasing aesthetics.
Human-Centric Applications: Many AI applications are explicitly designed to solve human problems, making it natural to prioritize human needs and perspectives.
Examples of Anthropocentric Bias in AI:
Here are several examples illustrating how anthropocentric bias can manifest in AI systems:
Natural Language Processing (NLP):
Bias in Language Models: Large language models (LLMs) like GPT-series are trained on massive datasets of text and code scraped from the internet. These datasets often contain biases related to gender, race, and other social categories. As a result, these models can perpetuate harmful stereotypes in their generated text.
Example: If prompted with "The doctor is...", the model might disproportionately generate "he" rather than "she," reflecting the historical underrepresentation of women in medicine. Similarly, prompts related to crime might elicit responses that unfairly associate certain racial groups with criminality.
Sentiment Analysis: Sentiment analysis tools are often trained on data labeled by humans based on their own understanding of emotions. However, emotions can be subjective and culturally influenced. An AI trained on Western expressions of emotion might misinterpret the sentiment expressed in non-Western cultures.
Image Recognition:
Bias in Object Detection: Object detection models can be trained on datasets that are biased in terms of the representation of different objects and demographics. For example, if a dataset contains mostly images of white people in leadership positions, the model might perform better at recognizing white people as leaders compared to people of color.
Example: Facial recognition systems have been shown to be less accurate in identifying individuals with darker skin tones, particularly women. This is due to the underrepresentation of these groups in the training datasets.
Aesthetic Judgement: AI systems designed to assess beauty or artistic merit often reflect human aesthetic preferences, which can be influenced by cultural norms and historical biases. An AI trained on Western art might undervalue or misinterpret art from other cultures.
Defining Rewards: In RL, agents learn to maximize rewards based on feedback from the environment. Defining these rewards requires injecting human values.
Example: Consider an AI designed to manage traffic flow. If the reward function primarily prioritizes minimizing travel time for individual drivers, the AI might neglect other important factors like pedestrian safety, pollution, and public transportation accessibility. This is because these factors are not explicitly included in the reward function based on anthropocentric priorities of driving time.
Autonomous Vehicles:
Ethical Dilemmas: Autonomous vehicles often face ethical dilemmas in situations where they must choose between different courses of action that could result in harm. The programming of these vehicles reflects human values and assumptions about what constitutes the least harmful outcome.
Example: In a scenario where a vehicle must choose between swerving into a pedestrian to avoid colliding with another car or maintaining course and hitting the car, the programming will inevitably reflect a human-defined hierarchy of values that prioritize certain lives over others.
Healthcare AI:
Diagnostic Bias: AI-powered diagnostic tools can perpetuate biases present in medical data. If a particular disease is underdiagnosed in a certain demographic, the AI might learn to overlook symptoms in that population, leading to misdiagnosis.
Example: If data on heart disease primarily comes from men, an AI diagnostic tool might be less accurate in detecting heart disease in women, who may present with different symptoms.
Consequences of Anthropocentric Bias in AI:
The consequences of anthropocentric bias in AI can be far-reaching and detrimental:
Perpetuation of Inequality: Biased AI systems can reinforce existing social inequalities, discriminating against marginalized groups in areas such as employment, education, and criminal justice.
Reduced Accuracy and Reliability: AI models trained on biased data may perform poorly on data from underrepresented groups, leading to inaccurate predictions and unreliable outcomes.
Erosion of Trust: If AI systems are perceived as unfair or biased, it can erode public trust in the technology and hinder its widespread adoption.
Limited Innovation: By focusing solely on human needs and perspectives, we may miss out on opportunities to develop AI systems that can solve problems in novel and unexpected ways.
Ethical Concerns: The use of biased AI systems raises serious ethical concerns about fairness, justice, and accountability.
Mitigating Anthropocentric Bias in AI:
Addressing anthropocentric bias in AI requires a multi-faceted approach that involves technical, ethical, and social considerations:
Data Diversity and Augmentation: Ensure that training datasets are diverse and representative of the population that the AI system will interact with. Data augmentation techniques can be used to address imbalances in the data.
Bias Detection and Mitigation Techniques: Employ techniques to detect and mitigate bias in AI models, such as adversarial debiasing, fairness-aware learning, and explainable AI (XAI) methods.
Human-in-the-Loop Design: Involve diverse stakeholders in the design and development of AI systems to ensure that different perspectives are considered. Human oversight and feedback can help to identify and correct biases in real-time.
Explainable AI (XAI): Develop AI systems that are transparent and explainable, allowing users to understand how decisions are made and identify potential biases. This can help to build trust and accountability.
Ethical Guidelines and Regulations: Establish ethical guidelines and regulations for the development and deployment of AI systems to ensure that they are used responsibly and fairly.
Critical Reflection: Foster a culture of critical reflection and awareness among AI developers, researchers, and policymakers to challenge anthropocentric assumptions and biases.
Interdisciplinary Collaboration: Encourage collaboration between AI researchers, ethicists, social scientists, and domain experts to address the complex ethical and social implications of AI.
Consider Non-Human Perspectives: While challenging, explore methods for incorporating non-human perspectives and values into AI design. This might involve studying animal behavior, ecological systems, or philosophical theories about the nature of intelligence and consciousness.
Beyond Anthropocentrism: Embracing a More Inclusive Future for AI:
Ultimately, addressing anthropocentric bias in AI requires a fundamental shift in perspective. We need to move beyond viewing AI solely as a tool for serving human needs and begin to recognize its potential to benefit the world in broader ways. This involves:
Acknowledging the limitations of human perspective: Recognizing that human understanding is inherently limited and that there are other valid ways of perceiving and interacting with the world.
Valuing diversity and inclusivity: Embracing diversity in all its forms – not only in terms of human demographics but also in terms of perspectives, values, and intelligences.
Promoting ecological awareness: Developing AI systems that are mindful of their impact on the environment and that contribute to a more sustainable future.
Exploring the potential of non-human intelligence: Investigating the diverse forms of intelligence that exist in the natural world and exploring how these insights can inform the development of AI.
By acknowledging and addressing anthropocentric bias, we can create AI systems that are fairer, more accurate, and more beneficial to all. This requires ongoing vigilance, critical reflection, and a commitment to building a more inclusive and equitable future for both humans and AI.
Comments