Task Transformation: How Modular and Reconfigurable Locomotion Supercharges Task Completion in Antetic AI
- Aki Kakko
- 22 hours ago
- 6 min read
The ability to adapt to changing environments and task requirements is a hallmark of intelligent systems. In Antetic AI, where swarms of agents collaborate to achieve complex goals, Modular and Reconfigurable Locomotion (MRL) emerges as a transformative capability. It allows individual agents to dynamically alter their physical form and mode of movement, enabling them to tackle a wider range of tasks and optimize their performance in diverse situations. This article explores the profound impact of MRL on task completion within Antetic AI, outlining its mechanisms, benefits, and illustrative applications.
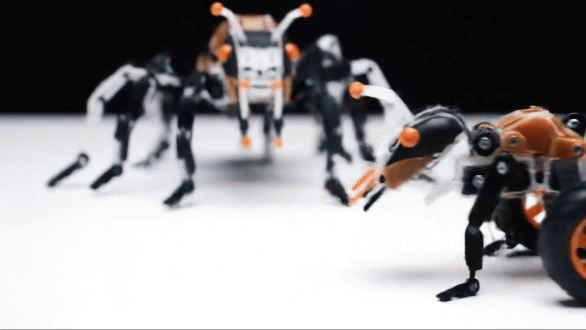
Beyond Fixed Forms: The Power of Embodied Adaptability
Traditional robots are often designed with a fixed morphology, limiting their versatility. MRL breaks free from this constraint by allowing agents to:
Change their Shape: Reconfiguring their physical structure to better suit the task at hand.
Change their Gait: Switching between different modes of locomotion (e.g., walking, rolling, climbing) to optimize movement across various terrains.
Change their Toolset: Attaching or detaching specialized tools to perform different actions.
Change their Sensory Configuration: Re-positioning sensors for optimal environment awareness.
Form Coalitions and Attach to other Robots: If another robot is better equipped for a specific task, the other AI can attach to it to amplify their performance.
This adaptability at the embodied level is critical for task completion in the often unpredictable environments where Antetic AI systems operate.
Mechanisms for Achieving Modular and Reconfigurable Locomotion
Several approaches can be used to implement MRL in Antetic AI systems:
Modular Robotics with Standardized Interfaces:
Concept: Agents are composed of interchangeable modules with standardized mechanical and electrical interfaces.
Mechanism: Agents can attach and detach modules to reconfigure their shape, gait, and toolset. This requires robust connection mechanisms, power distribution systems, and communication protocols.
Example: A cleaning robot could add a brush module for sweeping floors, a vacuum module for collecting debris, or a climbing module for scaling walls. The standardized interfaces allow modules to be swapped quickly and easily, without requiring specialized tools or skills.
Self-Reconfiguring Robots:
Concept: Robots are composed of multiple identical modules that can move relative to each other to change the robot's shape and function.
Mechanism: This involves developing robots with internal mechanisms that allow the modules to rearrange themselves into different configurations. The robots can then use these configurations to perform different tasks or navigate different terrains.
Example: A robot could transform from a snake-like configuration for navigating narrow passages to a legged configuration for climbing over obstacles.
Programmable Materials and Soft Robotics:
Concept: Use materials that can change their shape, stiffness, or other properties in response to external stimuli.
Mechanism: This involves developing robots made from materials that can be programmed to morph into different shapes or change their stiffness. The robots can then use these capabilities to adapt to changing environments and task requirements.
Example: A robot could use soft robotics to inflate or deflate different sections of its body, allowing it to squeeze through narrow gaps or grasp objects of different shapes.
Tool Caching and Swapping:
Concept: Agents can access a cache of specialized tools and swap them as needed to perform different tasks.
Mechanism: This requires a system for storing and retrieving tools, as well as mechanisms for attaching and detaching tools to the agent. The agent can then select the appropriate tool based on the current task requirements.
Example: A construction robot could retrieve a hammer for driving nails, a screwdriver for tightening screws, or a saw for cutting wood.
Cooperative Reconfiguration:
Concept: Agents can combine or attach to each other to form larger, more capable structures.
Mechanism: This requires agents to have compatible interfaces and the ability to coordinate their movements. The resulting structure can then perform tasks that would be impossible for a single agent.
Example: Multiple robots could combine to form a bridge to span a gap, a platform to lift heavy objects, or a larger robot with greater strength or sensing capabilities.
Impact of MRL on Task Completion in Antetic AI
MRL significantly enhances task completion in several ways:
Increased Versatility: MRL enables agents to perform a wider range of tasks, expanding the scope of the Antetic AI system.
Improved Efficiency: Agents can adapt their form and locomotion to optimize their performance for specific tasks and environments.
Enhanced Robustness: MRL allows agents to compensate for damage or component failures by reconfiguring their structure or task assignments.
Greater Autonomy: Agents can autonomously adapt to changing conditions without requiring human intervention.
Emergent Capabilities: The combination of MRL with distributed control can lead to the emergence of new and unexpected capabilities, as agents discover new ways to reconfigure themselves and cooperate.
Reduced Costs: One robots changing and taking on different roles to make the most of each task instead of many single use robots for each task
Illustrative Applications of MRL in Antetic AI
Robots can morph into small, agile forms to access tight spaces, then expand for efficient cleaning of large surfaces.
Specialized modules, like scrubbers, vacuums, and disinfectant applicators, can be dynamically attached or detached based on the surface and type of mess.
Robots can transform to navigate collapsed structures, minefields, and radiation zones.
Heavy-duty modules are attached for clearing debris, neutralizing explosives, and handling hazardous materials.
The robots combine to form protective barriers and large vehicles for moving hazardous material from these areas.
Search and Rescue:
Robots could transform from wheeled vehicles for rapid transit to legged robots for navigating rubble.
They could combine to form a larger robot capable of lifting heavy debris or detecting faint signals.
Modular cameras or sensors could be attached or re-positioned to find areas.
Environmental Remediation:
Robots could adapt their locomotion to navigate contaminated terrain, switching between walking, crawling, and swimming.
They could attach specialized tools for collecting samples, removing pollutants, or neutralizing hazardous materials.
Construction and Infrastructure Maintenance:
Robots could reconfigure themselves to fit into tight spaces, climb vertical surfaces, or manipulate heavy objects.
They could combine to form scaffolding, bridges, or other temporary structures.
Planetary Exploration:
Rovers could adapt their locomotion to traverse diverse terrain types, from smooth plains to rocky mountains.
They could deploy specialized instruments for analyzing soil samples, collecting atmospheric data, or building habitats.
Space Situational Awareness:
Using agile bots that can form to large structures with advanced and diverse sensors to make space exploration more robust. With one AI system, this makes for a streamlined approach at low cost.
Challenges and Future Directions
Realizing the full potential of MRL in Antetic AI requires addressing several challenges:
Developing robust and reliable modular components and connection mechanisms.
Creating efficient control algorithms that can coordinate the movement and reconfiguration of multiple modules.
Designing sensors that can accurately perceive the environment and provide feedback to the control system.
Developing new materials that are both lightweight and strong, allowing for the creation of more agile and adaptable robots.
Addressing the increased energy consumption associated with reconfiguration processes.
Creating clear security measures to prevent unauthorized robots or parts to hook on and harm the robot hivemind.
Future research will focus on:
Exploring new materials and manufacturing techniques for creating modular robots.
Developing AI algorithms that can automatically design and optimize robot configurations for different tasks.
Creating simulation platforms that can accurately model the dynamics of reconfigurable robots and their interactions with the environment.
A Future of Transformative Task Completion
Modular and Reconfigurable Locomotion represents a paradigm shift in the design and operation of Antetic AI systems. By empowering agents to dynamically adapt their physical form and mode of movement, we can create AI swarms that are more versatile, efficient, robust, and autonomous than ever before. As we continue to explore the potential of this transformative technology, we can expect to see MRL play an increasingly important role in shaping the future of robotics, exploration, and artificial intelligence, enabling AI systems to tackle increasingly complex and challenging tasks in a wide range of environments. The key is to create AI embodiments that are not fixed and static, but dynamic and adaptable, capable of transforming themselves to meet the demands of a constantly changing world. This is the future of task completion in Antetic AI - a future defined by flexibility, innovation, and the power of embodied intelligence.
Comments