Investing involves a myriad of decisions based on assessments of risks, rewards, and comparisons between different options. An investor might wonder: “What would have been the outcome had I chosen another investment?” Answering such questions can be challenging due to confounding factors. This is where Propensity Score Matching (PSM) comes in. PSM is a statistical method used to reduce bias by equating groups based on these confounding factors.
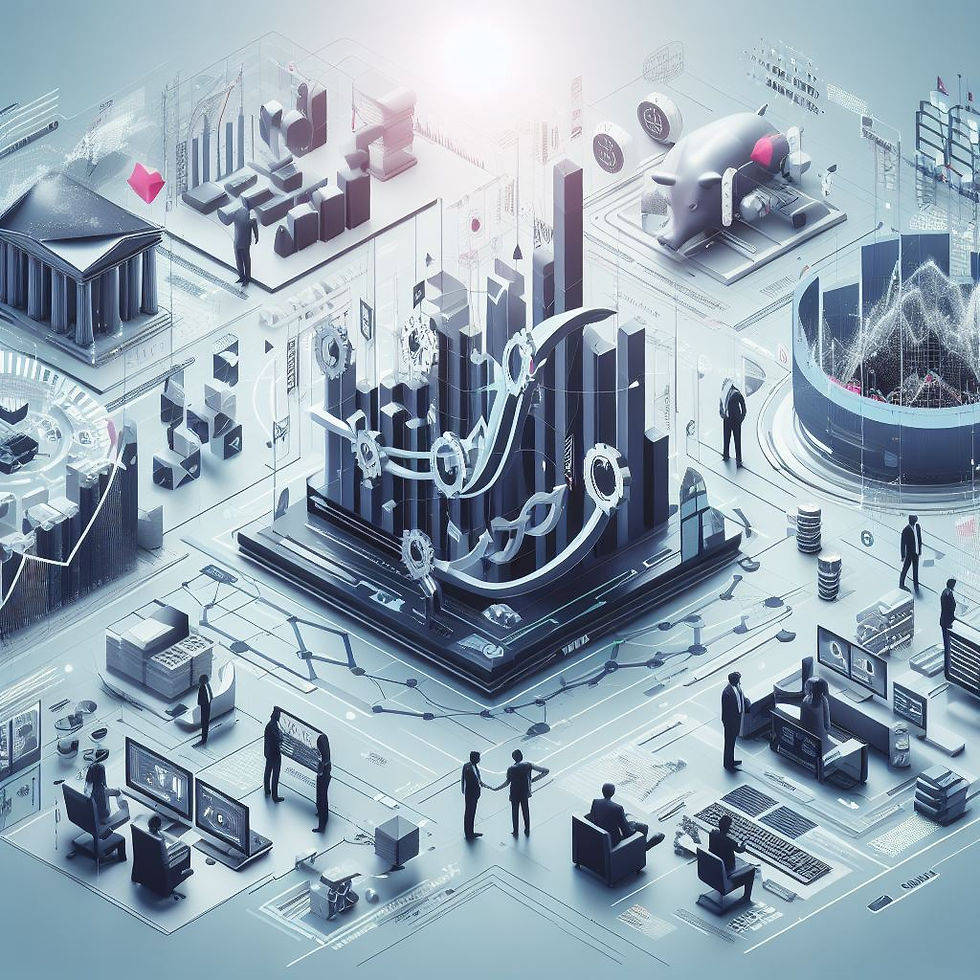
What is Propensity Score Matching (PSM)?
PSM is a method used to estimate causal effects by comparing treatment and control groups in observational studies. It aims to mimic the random assignment in an experimental design. The propensity score is the probability of treatment assignment conditional on observed baseline characteristics. Once we have these scores, we can match treated units to untreated units based on their propensity scores, allowing us to compare like with like.
Why is it Relevant to Investors?
For investors, PSM can be beneficial in several ways:
Evaluating the impact of an investment decision on portfolio returns, by comparing the treated group (those who made the investment) with a matched control group (those who didn’t).
Estimating the effect of corporate actions (e.g., mergers, restructuring) on stock prices.
Assessing the influence of macroeconomic policies or events on market indices or asset classes.
How does PSM Work?
PSM involves the following steps:
Estimation of Propensity Scores: Using logistic regression, estimate the probability that each unit (e.g., investment, company) receives the treatment based on observed characteristics.
Matching: Match treatment and control units based on their propensity scores. Several methods exist, such as:
Nearest neighbor matching: Matches a treated unit to a control unit with the closest propensity score.
Caliper matching: Similar to nearest neighbor, but only matches if the difference in propensity scores is below a certain threshold.
Stratification matching: Divides the propensity score into intervals, and ensures an equal number of treated and control units within each interval.
Outcome Analysis: Compare outcomes (e.g., returns, stock prices) between the matched treatment and control groups to estimate the causal effect.
Advanced Matching Techniques
Beyond the basic techniques, there are more advanced matching methods to consider:
Kernel Matching: Uses weighted averages of all controls to construct the counterfactual outcome, emphasizing controls with scores close to the treated units.
Radius Matching: A variation of caliper matching, it matches each treated unit to controls within a specified radius of the propensity score.
Multiple/Many-to-One Matching: In situations with fewer treated units, multiple control units can be matched to a single treated unit.
Mechanics of PSM
When thinking of PSM, visualize it as a 'balancing act'. PSM aims to balance the distribution of observed confounding variables between the treated and control groups, thus ensuring they are statistically similar.
Covariates: These are the observed characteristics or features used in the matching process. The success of PSM hinges on the thorough selection of relevant covariates.
Overlap Assumption: For PSM to work, there must be substantial overlap in the propensity scores of both groups, known as the 'common support' region. Without this, the matching process may be unreliable.
Example in an Investment Scenario:
Consider an investor interested in understanding the impact of a company's transition to sustainable practices on its stock price. The challenge is that companies choosing to go green might be inherently different from those that don’t. PSM can help.
Steps:
Defining Treatment and Control: Companies transitioning to green practices are the 'treatment' group. Those that aren’t are the 'control'.
Estimating Propensity Scores: Use logistic regression with factors like company size, sector, past profitability, etc., as predictors. The outcome is the binary decision (transitioned to green practices or not).
Matching: Use one of the matching techniques, e.g., nearest neighbor matching, to find control companies for each treated company.
Analysis: Compare stock price performance between the matched treatment and control groups over a specified time frame.
Conclusion: The difference in performance provides an estimate of the impact of transitioning to green practices on stock prices.
Applications in Investment Analysis
Evaluating Investment Strategies: Suppose an investment firm introduces a novel algorithmic trading strategy. They could use PSM to compare the returns of portfolios that employed this strategy against those that didn't, matching on factors like initial capital, historical volatility, and sector allocations.
Macro-Level Analysis: PSM could be instrumental in examining the effect of macroeconomic changes on sectors or stocks. For instance, when a country introduces tax benefits for renewable energy firms, PSM can help ascertain the policy's effect on stock prices by matching treated firms (those benefiting from the policy) with similar untreated firms.
Private Equity and Venture Capital: In VC or PE scenarios, PSM can gauge the impact of funding or mentorship programs. By matching funded startups with unfunded ones based on attributes like industry, founder experience, and product stage, investors can better understand the true value-add of their intervention.
Considerations for Robust Application
Choosing Relevant Covariates: Critical to PSM's success is the careful selection of covariates. Ignoring key covariates can lead to 'hidden bias'.
Data Quality: The accuracy of PSM relies heavily on the quality of data. Missing data or incorrect observations can distort the propensity scores and the resulting matches.
Sensitivity Analysis: Once PSM is performed, it's wise for investors to conduct sensitivity analyses. This checks how robust the findings are to potential deviations from the assumed model.
Limitations of PSM:
Unobserved Confounding: PSM only controls for observed confounders. If there are unobserved factors influencing both treatment and outcome, bias might remain.
Model Dependency: The propensity score's accuracy depends on the correct specification of the logistic regression model.
Quality of Matches: If there aren’t good matches for each treated unit, PSM might not reduce bias sufficiently.
While PSM offers a potent tool for investors, its efficacy relies on its correct application. By understanding its intricacies and being mindful of its limitations, investors can harness PSM to derive valuable insights, leading to more informed and strategic decisions in the ever-evolving financial landscape.
Join our Discord: https://discord.gg/SyVKHbd6 to #OpenSourceWallStreet
コメント