Principal Component Analysis (PCA) is a powerful statistical technique used for dimensionality reduction. It allows researchers, analysts, and investors to transform a set of correlated variables into a smaller set of uncorrelated variables, known as principal components. In the investment world, PCA can provide clarity in understanding complex datasets and facilitate better decision-making.
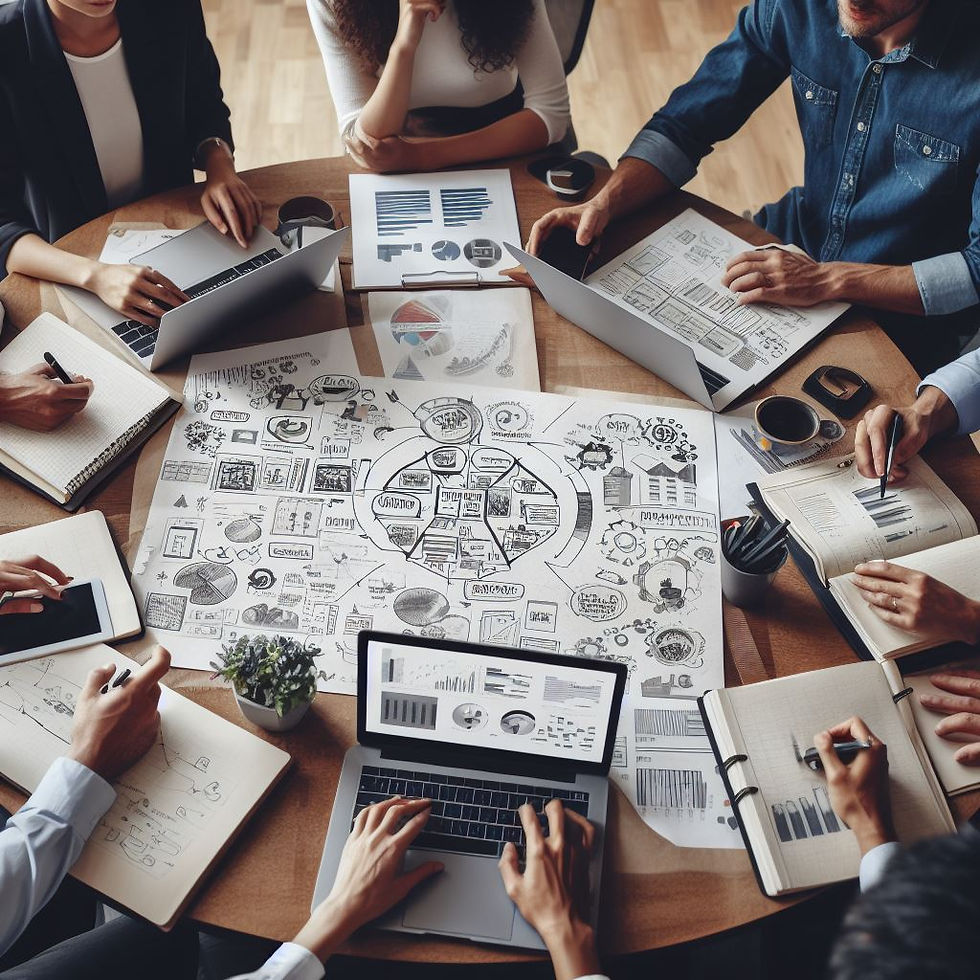
Why is PCA Relevant to Investors?
Portfolio Diversification: Diversifying investments is a primary strategy to manage risk. PCA can help in determining asset combinations that have low correlations, providing true diversification.
Factor Analysis: For investors who adopt factor-based investing strategies, PCA can identify which factors (like value, size, or momentum) are the most relevant and influential.
Noise Reduction: PCA can reduce the ‘noise’ in financial data, enabling investors to focus on the most significant variables.
How Does PCA Work?
PCA starts by calculating the covariance matrix for the data. This matrix provides a measure of how different variables change relative to each other. Then, PCA identifies the principal components through eigen decomposition, sorting them in descending order based on their explained variance.
Example with Stocks:
Imagine you're analyzing three tech stocks: Apple (AAPL), Google (GOOGL), and Microsoft (MSFT). Over several years, you have monthly return data for each stock. This data is likely correlated since the tech industry shares many commonalities. Using PCA, you can find principal components that represent the combined variance of these stocks. The first principal component might capture the overall tech industry's movement, while subsequent components might capture the specificities of each stock. By doing so, you’ve reduced the dimensionality of your data. Instead of focusing on three stocks individually, you might only need to consider one or two principal components to understand the majority of the variance in your dataset.
Practical Steps for Using PCA in Investing:
Data Standardization: Before applying PCA, ensure all variables are standardized to have a mean of 0 and a standard deviation of 1.
Calculate the Covariance Matrix: This will capture the relationships between variables.
Eigen Decomposition: Derive eigenvalues and eigenvectors from the covariance matrix. The eigenvectors represent the principal components, while the eigenvalues indicate their magnitude.
Sort Components by Variance: Rank the components based on the variance they explain. The first principal component will usually capture the most variance.
Data Projection: Use the top principal components to transform the original dataset into a lower-dimensional one.
Benefits for Investors:
Enhanced Portfolio Optimization: PCA allows for improved asset allocation by concentrating on combinations that offer genuine diversification.
Clearer Insights: It simplifies data, offering a clearer view of underlying patterns.
Better Decision Making: By focusing on the most influential factors, investors can make more informed decisions.
Limitations of PCA:
Linear Assumption: PCA assumes linear relationships among variables. If relationships are nonlinear, PCA might not capture the nuances.
Variance ≠ Information: The first principal components explain the most variance, but they might not always carry the most meaningful information.
Interpretability: Principal components may not always have a clear, intuitive interpretation in the real world.
Principal Component Analysis is a valuable tool for investors, offering a systematic way to reduce complex data into its most essential components. By understanding and leveraging PCA, investors can enhance portfolio diversification, gain clearer insights from financial datasets, and make more informed investment decisions. As with any technique, it's crucial to understand its limitations and use it judiciously in the context of broader investment strategies.
Commentaires