Longitudinal data analysis plays a crucial role in causal inference, especially in fields such as economics, epidemiology, and social sciences. This type of analysis involves studying the same subjects repeatedly over time, allowing researchers to observe changes and make inferences about causality. Here's a detailed look at how longitudinal data analysis is used in causal inference, with examples to illustrate key points.
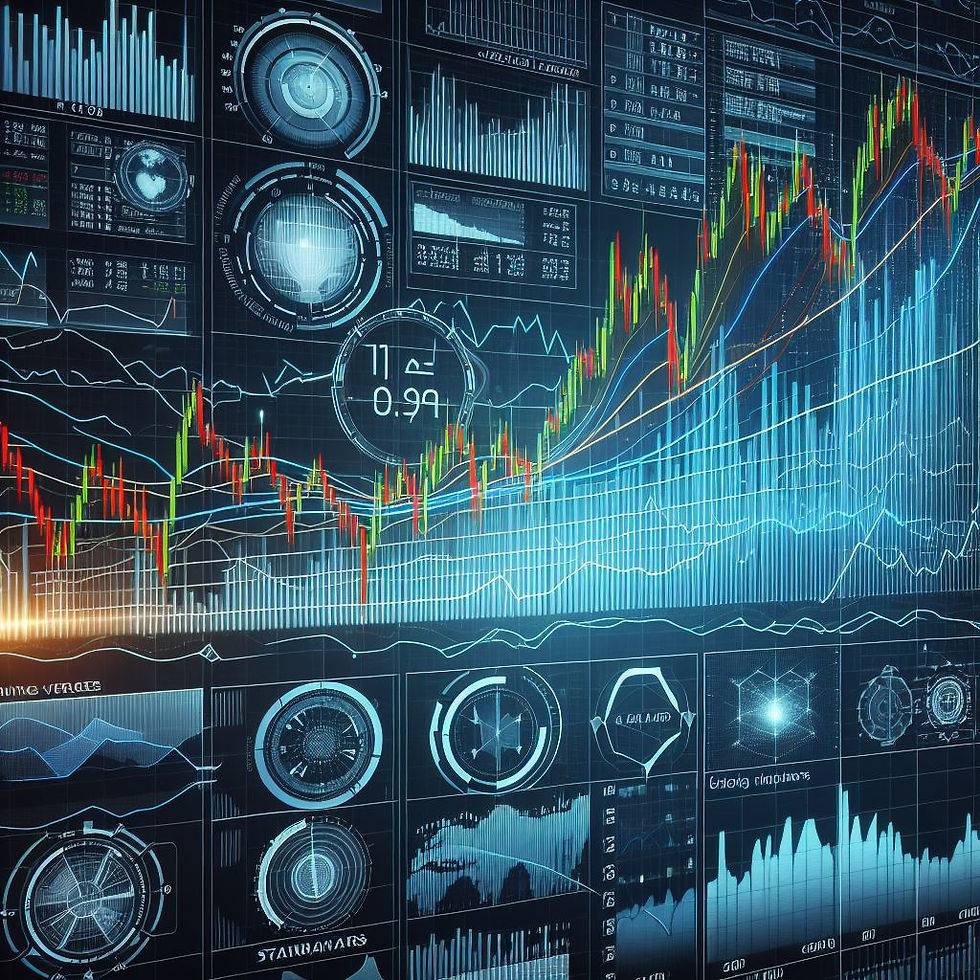
Understanding Longitudinal Data Analysis
Longitudinal data analysis involves the examination of data collected from the same subjects at multiple points in time. This approach is essential for understanding how variables evolve over time and their causal relationships.
Advantages Over Cross-Sectional Studies
Unlike cross-sectional studies, which provide a snapshot at a single point in time, longitudinal studies can track changes, identify trends, and establish temporal sequences essential for causal inference.
Key Concepts in Longitudinal Data Analysis
Time-Varying and Time-Invariant Variables: In longitudinal studies, researchers deal with time-varying variables (that change over time, like income level) and time-invariant variables (constant, like gender).
Fixed and Random Effects Models: These statistical models are used to analyze longitudinal data. Fixed effects models control for time-invariant unobserved heterogeneity, while random effects models assume random variation across subjects.
Longitudinal Data Analysis in Causal Inference
Establishing Temporal Precedence: Longitudinal data is essential to establish that the cause precedes the effect, a key criterion for causal inference.
Controlling for Confounders: By tracking subjects over time, researchers can control for unobserved confounders, which might otherwise bias the results.
Understanding Dynamics of Change: This analysis helps in understanding how changes in one variable lead to changes in another over time, providing a dynamic perspective of causality.
Examples in Various Fields
Economics: Examining the impact of education policies on income levels over decades, by tracking a cohort of individuals from school through their careers.
Healthcare: Assessing the long-term effects of a new medication on patient health, observing side effects and outcomes over several years.
Social Sciences: Studying the impact of early childhood interventions on adult outcomes by following a group of individuals from childhood into adulthood.
Real Estate Investment: An investor tracks property values in a specific area over 20 years, analyzing the impact of urban development projects, demographic shifts, and economic policies on real estate prices.
Technology Sector Analysis: By examining the rise of a tech company over a decade, including product launches and market expansions, investors can understand the factors contributing to its growth and potential future trajectory.
Impact of Economic Recession: Studying how different industries fared during and after a recession, investors can understand which sectors are more resilient and adjust their portfolios accordingly.
Methodological Considerations
Data Collection Challenges: Longitudinal studies require consistent and repeated data collection, which can be resource-intensive and prone to attrition bias.
Statistical Complexity: Analyzing longitudinal data often requires advanced statistical techniques and software, and a deep understanding of the models used.
Interpreting Results: It's crucial to account for the complexity of real-world data, ensuring that causal inferences drawn are valid and reliable.
Practical Implications for Investors
Investors can leverage longitudinal data analysis in several ways:
Risk Assessment: By understanding how variables change over time, investors can better assess the risks associated with different investments. For example, tracking the performance of a company over several years can reveal patterns that might not be evident in a single fiscal report.
Market Trends Analysis: Longitudinal studies can help investors identify long-term market trends, enabling them to make more informed decisions about where to allocate their resources.
Evaluating Policy Impact: When a new policy is implemented, its effect on markets or specific sectors can be effectively gauged through longitudinal analysis. This is particularly relevant for regulatory changes, tax reforms, or shifts in trade policies.
Challenges and Solutions
Data Availability and Quality: One of the significant challenges in longitudinal analysis is obtaining high-quality, consistent data over extended periods. Investors need to rely on credible sources and may need to invest in data collection and management tools.
Complexity in Analysis: Longitudinal data analysis can be technically challenging. Investors may need to collaborate with statisticians or use advanced analytics software to correctly interpret the data.
Keeping Up with Changes: As markets and industries evolve rapidly, it's essential to continually update and revise longitudinal analyses to keep them relevant.
Future Directions
Integration with Big Data and AI: Merging longitudinal data analysis with big data analytics and AI can provide more profound insights and predictive analytics, helping investors make more nuanced decisions.
Real-Time Data Tracking: With advancements in technology, real-time data tracking can complement traditional longitudinal studies, providing a more dynamic and updated view of market changes.
Cross-Industry Analysis: Investors can benefit from longitudinal analyses that span across industries, providing a holistic view of the market dynamics and identifying interdependencies between different sectors.
For investors, longitudinal data analysis is not just a statistical tool but a strategic asset that can significantly enhance decision-making. By understanding the temporal dynamics of the markets, the impact of various factors over time, and adapting to the methodological challenges, investors can gain a competitive edge and achieve long-term success in their investments. As technology continues to evolve, the potential for more advanced and insightful longitudinal analyses in the investment world is vast and promising.
Comments