In the fast-paced world of artificial intelligence, Inverse Reinforcement Learning (IRL) has emerged as a novel approach to understanding the preferences and decisions of intelligent agents. But what does this mean for investors? In this article, we will delve deep into the world of IRL, understand its fundamentals, and explore how it can be a game-changer for investment strategies.
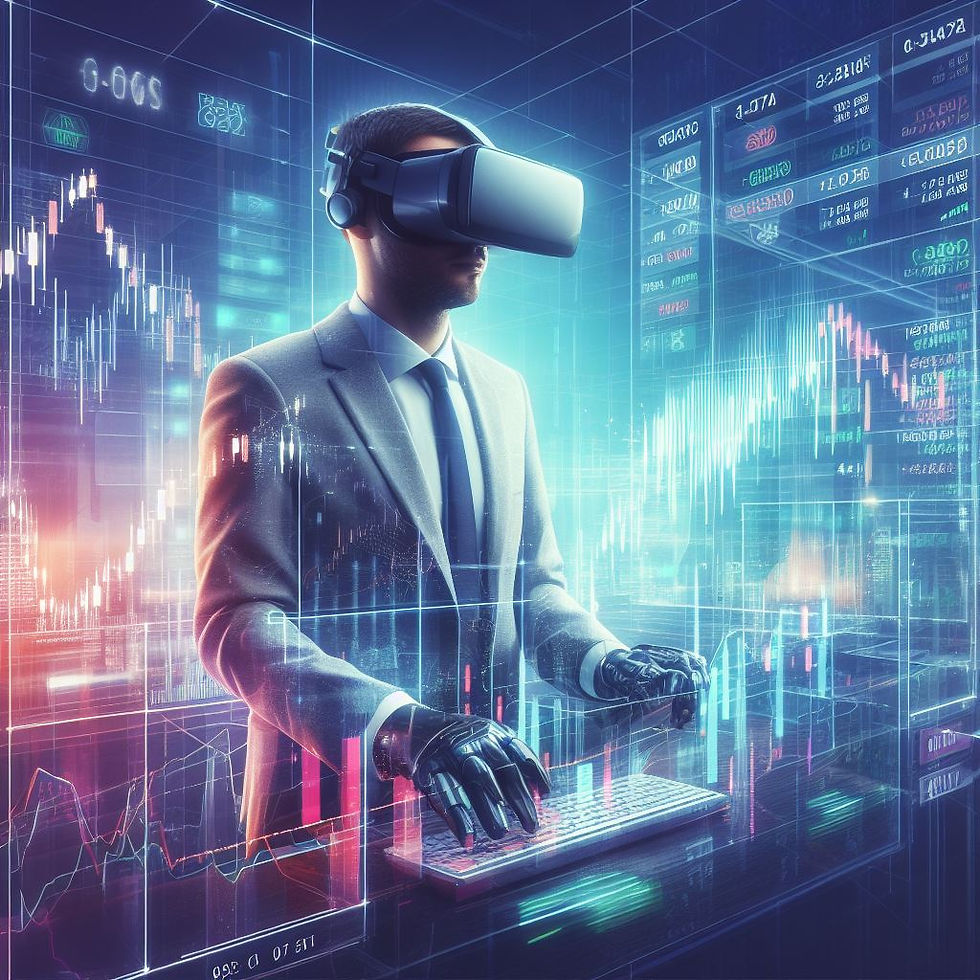
What is Inverse Reinforcement Learning?
At its core, Reinforcement Learning (RL) is a type of machine learning where an agent learns to make decisions by interacting with an environment. The agent receives rewards for desirable actions and penalties for undesirable ones. Over time, the agent learns to take actions that maximize its cumulative reward. Inverse Reinforcement Learning flips this process. Instead of learning from rewards to derive an optimal policy (or action strategy), IRL seeks to understand the reward function itself, given a set of observed optimal actions. In simpler terms, while RL asks, "Given the rewards, what should I do?", IRL asks, "Seeing what was done, what were they optimizing for?".
The IRL Process:
The typical IRL process involves several steps:
Observation: Collect data on the agent's behavior in different scenarios.
Modeling: Use this data to create a model that represents the decision-making process of the agent.
Inference: Deduce the likely reward function or preference structure that could lead to the observed behaviors.
Validation: Test the inferred reward function with new data to check the accuracy of the IRL model.
Algorithms and Techniques:
Various algorithms underpin IRL, such as:
Linear IRL: Assumes the reward function can be represented as a linear combination of features.
Maximum Entropy IRL: Finds the reward function by considering the most probable behavior given the observed actions and avoids overfitting by maximizing the entropy.
Deep IRL: Uses deep neural networks to represent complex, non-linear reward functions.
Why is IRL important for investors?
Understanding Market Dynamics: IRL can be used to infer the objectives or preferences of market participants based on their observed actions. This understanding can offer unique insights into market trends, especially in complex scenarios where traditional models may falter.
Algorithmic Trading: Trading strategies often involve RL algorithms that are trained with historical data to optimize returns. By applying IRL, investors can uncover the implicit reward functions used by other traders, potentially allowing for improved strategies that anticipate market moves.
Risk Management: By deciphering the underlying motivations and objectives of market participants, IRL can also help in identifying systemic risks that might not be evident from traditional data analysis.
Enhanced Portfolio Management: Advanced AI-driven portfolio management systems, known as robo-advisors, can integrate IRL to optimize asset allocation. By analyzing investor behavior, IRL can deduce underlying preferences (like risk tolerance or investment horizons) that might not be explicitly stated, leading to more tailored investment strategies.
Behavioral Finance Insights: IRL can be a powerful tool in the realm of behavioral finance. Traditional models often assume rational behavior, but in reality, emotions, biases, and other factors can drive financial decisions. IRL can provide a deeper understanding of these behavioral nuances by analyzing real-world financial actions.
Financial Crime Detection: Suspicious trading patterns or unusual financial behaviors can be scrutinized using IRL. By understanding the "normal" reward functions of traders or financial entities, anomalies can be more readily detected, aiding in the identification of potential fraud or money laundering activities.
Real-world examples:
Decoding Financial Behaviors: Consider a hedge fund that notices a specific pattern of trading from one of its competitors. Using IRL, the fund might deduce the competitor's valuation model or risk-assessment strategy, giving the fund an edge in anticipating future trades.
Consumer Preference Modeling: Outside of pure finance, IRL can be employed in the broader context of economic behaviors. For instance, by observing the purchase decisions of consumers, companies might use IRL to infer underlying consumer preferences or values, aiding in product development or marketing strategies.
Macroeconomic Analysis: At a higher level, central banks or financial institutions could use IRL to interpret actions taken by economies, deducing underlying economic health indicators or predicting future fiscal/monetary policy changes.
The Future of IRL in Finance:
As data becomes more abundant and computational capabilities grow, the applications of IRL are set to expand. Potential future applications include:
Personalized Banking: Banks could use IRL to tailor services to individual customers based on their transaction histories, offering customized loan rates, savings plans, or even personalized financial advice.
Market Sentiment Analysis: By analyzing collective actions of traders and investors, IRL can infer prevailing market sentiments, aiding in macro-level investment decisions.
Mergers and Acquisitions Strategy: Firms could employ IRL to infer the strategic objectives of competitors, enabling better positioning in negotiations or identifying potential acquisition targets.
Investing in IRL-Driven Startups:
For investors looking at the tech landscape, startups or companies incorporating IRL into their technologies present promising opportunities. These entities are well-positioned to revolutionize industries, from finance and healthcare to entertainment and logistics. For example, a startup that offers IRL-based solutions to e-commerce platforms to better understand consumer choices can significantly improve sales forecasts and inventory management.
Potential Risks:
Like any investment, there are associated risks:
Overfitting: An IRL model that's too closely tailored to past data might perform poorly with future, unseen data.
Model Interpretability: IRL models, especially deep learning variants, can be complex and difficult to interpret, which may obscure understanding and trust.
Data Dependence: IRL's efficacy is dependent on high-quality, representative data. Poor or biased data can lead to skewed inferences.
Ethical Implications: The use of IRL in deducing preferences or strategies might raise privacy concerns. Transparent data handling and usage policies are crucial.
Regulatory Challenges: As with any advanced AI technique, there's potential for regulatory scrutiny, especially if IRL-driven strategies dominate market dynamics or if there's potential misuse.
Skill Gap: The complexity of IRL means a skill gap exists. Investments in training and research are vital to leverage its full potential.
Inverse Reinforcement Learning stands at the crossroads of cutting-edge AI and the intricate dynamics of financial decision-making. Its ability to discern underlying preferences and motivations from observed actions offers transformative insights, whether in portfolio management, behavioral finance, or market sentiment analysis. For investors, embracing IRL means not just staying ahead of the curve, but shaping it. However, as with all powerful tools, it comes with ethical, regulatory, and skill-related challenges that must be navigated judiciously. In the evolving dance of technology and finance, IRL promises to be a significant player, offering those who understand its nuances a distinct advantage in the investment arena.
Σχόλια