Illuminating the Black Box: Global Workspace Theory and its Role in Artificial Intelligence
- Aki Kakko
- Apr 9
- 6 min read
AI strives to create systems capable of intelligent behavior, often drawing inspiration from the most powerful intelligent system known: the human brain and mind. One influential cognitive mode is Global Workspace Theory (GWT), originally proposed by cognitive psychologist Bernard Baars. GWT offers a framework for understanding consciousness, attention, and information integration – concepts crucial for developing more flexible, robust, and perhaps even understandable AI systems. This article is about Global Workspace Theory and its relevance to AI, examines how it can be implemented computationally, provides illustrative examples, and discusses the associated challenges and future potential.
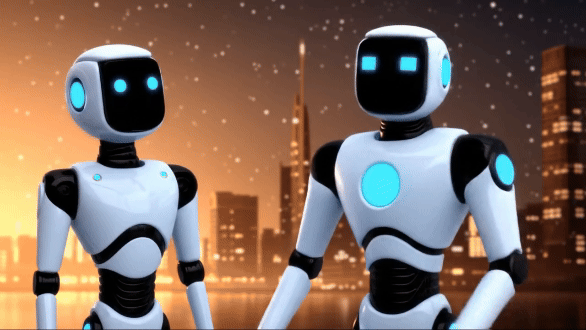
What is Global Workspace Theory (GWT)?
Bernard Baars introduced GWT in the 1980s as a cognitive architecture attempting to explain the role and function of consciousness. It uses the metaphor of a "theater of consciousness":
The Stage: Represents working memory – the very limited capacity system holding the current contents of consciousness. Only a small amount of information can be "on stage" at any given moment.
The Spotlight of Attention: Controls what information gets onto the stage. Attention selects the most relevant, salient, or urgent information from various potential inputs.
The Audience: Consists of a vast array of specialized, unconscious, parallel processors or modules in the brain (or an AI system). These modules handle specific tasks like visual perception, language processing, memory retrieval, motor control, etc. They operate largely independently and in parallel.
Broadcasting: Once information is on the stage (i.e., conscious), it is "broadcast" globally to the entire audience of unconscious processors. This allows diverse modules to receive and potentially react to the conscious information, facilitating coordination, learning, and problem-solving.
Contexts (Behind the Scenes): These are unconscious systems that shape conscious experience without being conscious themselves. They include structures that direct the spotlight of attention, set goals, manage expectations, and frame the current situation (e.g., long-term memory structures, intrinsic motivations).
In essence, GWT posits that consciousness acts as a central information exchange, allowing otherwise isolated specialist modules to cooperate, share information, and contribute to complex, serial tasks that require integrating diverse inputs. What we experience as the "stream of consciousness" is the sequence of information pieces briefly occupying the global workspace stage.
Why Apply GWT to AI?
While AI doesn't necessarily need to replicate human consciousness perfectly, the functional aspects described by GWT offer solutions to several key challenges in AI development:
Information Integration: Modern AI systems often consist of multiple specialized components (e.g., a vision module, a natural language processing module, a planning module). GWT provides a blueprint for how these diverse modules can effectively share information and coordinate their activities through a central "workspace."
Selective Attention: In complex environments, AI systems are bombarded with data. GWT's "spotlight" mechanism provides a model for focusing computational resources on the most relevant information, ignoring distractions, and prioritizing tasks.
Flexible Control and Problem Solving: Broadcasting information globally allows the system to recruit relevant modules dynamically to address novel situations or complex problems that no single module can solve alone.
Error Detection and Handling: When a mismatch or error is detected by a specialized module (e.g., prediction vs. reality), bringing this error signal into the global workspace allows the entire system to be notified, potentially triggering corrective actions, learning, or replanning.
Reportability and Explainability (XAI): The contents of the global workspace are, by definition, the information available for reporting. An AI based on GWT could potentially "report" on its current focus and the information it's using for decision-making, enhancing transparency.
Serial Processing from Parallel Systems: Like the brain, AI can leverage massive parallelism for low-level processing. GWT explains how a functionally serial "thought process" can emerge from this parallel substrate, enabling coherent sequences of actions or reasoning.
Implementing GWT in AI Architectures: Examples
Translating GWT into a computational framework involves creating an architecture where specialized modules communicate via a central, limited-capacity "workspace" regulated by an attention mechanism.
Conceptual Architecture:
Modules (The Audience): These could be neural networks, symbolic reasoners, algorithms, or other software components, each specializing in a task (e.g., object detection, sentence parsing, path planning, database querying).
Global Workspace (The Stage): A shared data structure (like a "blackboard" in older AI systems) with limited capacity. It holds the currently attended information (e.g., representations of perceived objects, parsed sentences, current goals, detected anomalies).
Attention Mechanism (The Spotlight): A component that evaluates potential inputs from various modules based on factors like salience (e.g., unexpectedness, intensity), relevance to current goals, and explicit directives. It selects which information enters the workspace.
Broadcasting Mechanism: A system ensuring that information placed in the workspace is made available to all (or a relevant subset of) other modules.
Context Systems: Modules or data structures representing goals, long-term memory associations, and current task context, influencing the attention mechanism.
Example: An Autonomous Robot Navigating a Room
Scenario: A robot needs to find and pick up a specific object (e.g., a red ball) in a cluttered room.
Modules:
VisionModule: Detects shapes, colors, textures. Operates in parallel across the visual field.
ObjectRecognitionModule: Identifies objects based on visual features.
SLAMModule (Simultaneous Localization and Mapping): Builds a map of the environment and tracks the robot's position.
PathPlanningModule: Calculates routes to specific locations.
MotorControlModule: Executes movements (wheels, arm).
GoalManagementModule: Holds the current goal ("Find Red Ball", "Pick Up Ball"). Influences attention.
ObstacleDetectionModule: Specifically looks for potential collision hazards.
GWT in Action:
Input & Competition: VisionModule detects multiple items. ObjectRecognitionModule identifies some (e.g., "Chair", "Blue Box", "Red Sphere"). ObstacleDetectionModule flags a nearby table leg. All these potential percepts compete for entry into the workspace.
Attention (Spotlight): The GoalManagementModule biases attention towards "red" and "ball-shaped" objects. The ObstacleDetectionModule's output has high intrinsic salience (potential danger). Let's say the "Red Sphere" is most relevant to the goal and sufficiently salient. The attention mechanism selects the representation of the "Red Sphere at coordinates (x,y)".
Workspace (Stage): The representation "Red Sphere at (x,y)" enters the global workspace.
Broadcasting: This information is broadcast to all modules.
Coordinated Response:
PathPlanningModule receives the location (x,y) and calculates a path.
MotorControlModule receives the path and starts moving the robot.
GoalManagementModule updates the state (e.g., "Ball located, moving towards it").
SLAMModule uses the movement commands and sensor data to update the map and robot pose.
If, during movement, ObstacleDetectionModule detects an imminent collision, this high-salience signal might win the next attentional competition, enter the workspace ("Obstacle ahead!"), be broadcast, and cause MotorControlModule to stop and PathPlanningModule to recalculate the path.
Challenges and Limitations
Despite its appeal, implementing GWT in AI faces challenges:
Defining Consciousness: GWT provides a functional model. Implementing it doesn't automatically grant an AI subjective experience or sentience (qualia). The focus is on functional similarity, not ontological identity.
Scalability: The broadcasting mechanism could become a bottleneck in systems with thousands or millions of modules. Efficient implementations require careful design, perhaps using selective or hierarchical broadcasting.
The Nature of the Workspace: What form should information take in the workspace? Symbolic representations? Sub-symbolic patterns (like neural activations)? How is capacity limited?
Implementing Attention: Designing effective computational attention mechanisms that balance goal-directed focus and sensitivity to unexpected salient events is complex. How is relevance calculated? How is competition resolved?
Module Design: GWT assumes the existence of specialized modules. Designing, training, and coordinating these modules remains a significant AI engineering task.
Learning: How does a GWT-based system learn? While broadcasting facilitates learning by distributing relevant information (like error signals or rewards), the specific learning mechanisms within modules or for tuning attention need to be defined.
Current Research and Future Directions
Several AI architectures have been explicitly inspired by GWT, such as LIDA (Learning Intelligent Distribution Agent) model. Research in areas like reinforcement learning (attention mechanisms), multi-agent systems (coordination), and modular deep learning implicitly touches upon GWT principles. Future directions include:
Hybrid Architectures: Combining GWT principles with deep learning modules for perception and pattern recognition, and symbolic reasoning modules for high-level planning.
AGI Development: GWT provides a plausible cognitive architecture that could contribute to building Artificial General Intelligence (AGI) capable of flexible, context-aware behavior across diverse domains.
Explainable AI (XAI): Leveraging the workspace content to provide users with insight into the AI's current focus and reasoning process.
Robustness and Adaptability: Using the global broadcast of anomalies or novel situations to trigger system-wide adaptation and learning, making AI less brittle.
Global Workspace Theory offers more than just a metaphor for AI; it provides a functional blueprint for integrating information, managing attention, and coordinating diverse computational processes. While challenges remain in its implementation and it doesn't magically solve the "hard problem" of subjective consciousness, GWT provides valuable inspiration for designing AI systems that are more integrated, flexible, and potentially more understandable. By illuminating the workspace where information is globally shared and processed, GWT concepts are helping to guide the development of the next generation of intelligent machines, moving us closer to AI that can handle the complexity and dynamism of the real world in a more brain-like manner.
Comentários