The Graphical Lasso (GL) is a statistical method that is utilized to estimate sparse inverse covariance matrices, which are pivotal for understanding relationships between variables in high-dimensional datasets. When applied to causal inference, the aim is to deduce the causal relationships among these variables. Graphical Lasso is a tool used by experts to understand relationships in large amounts of data. It helps investors see how different factors are connected, which is vital for making smart investment choices.
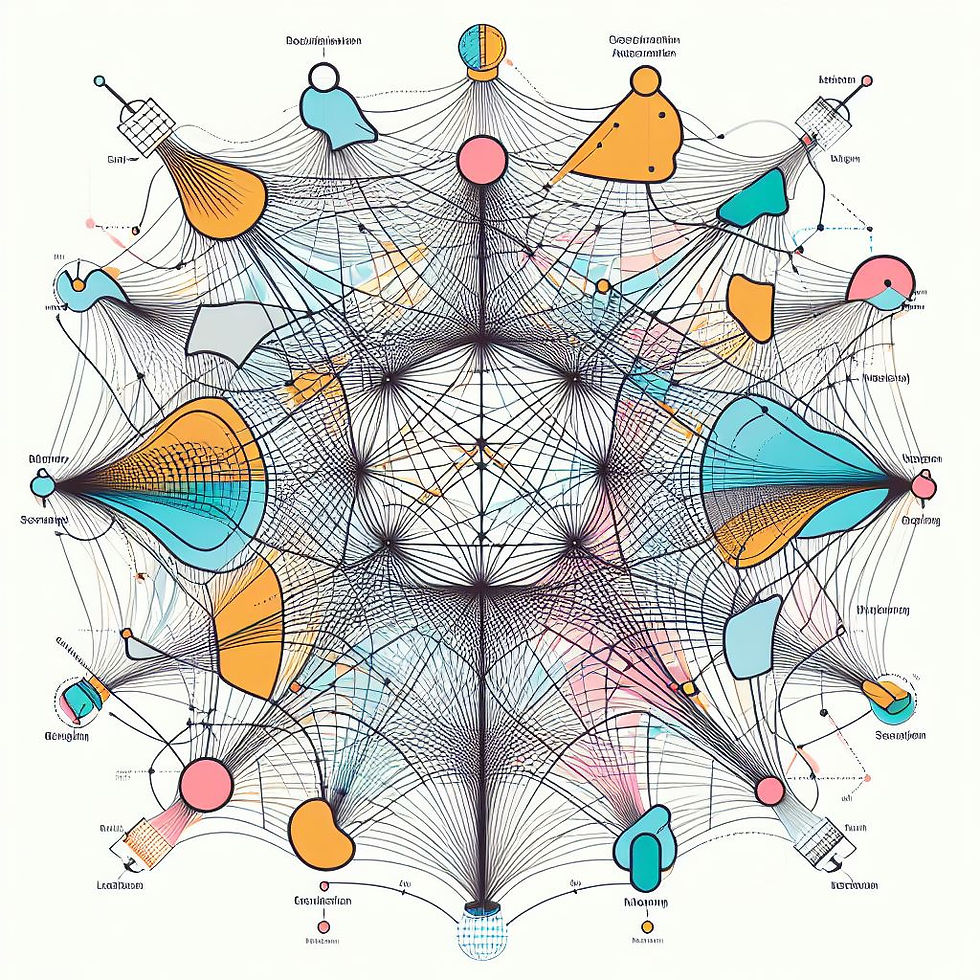
Different Methods & Their Uses:
Directed Adaptive Graphical Lasso: Different from the typical Graphical Lasso methods used for undirected graph structure learning, the Directed Adaptive Graphical Lasso focuses on a more challenging topic of directed graph structure inference. It discards the assumption of knowing the nodes' order and allows for a more generalized sparse learning framework for Directed Acyclic Graph (DAG) causality. The main objective of this method is to estimate a non-negative sparse precision matrix from empirical data.
Outcome-adaptive Lasso: This is an extension of the traditional Lasso method and is especially pertinent when dealing with causal inference from observational data. An essential assumption in this method is that all confounders affecting the relationship between treatment and outcome are measured and included in the model.
Multi-task Attributed Graphical Lasso (MAGL): This method is designed to address the limitations of the Multi-task Graphical Lasso, which is used for estimating graphs that share an identical set of variables. MAGL specifically aims to learn graphs that have both observations and attributes jointly, offering a solution when tasks involve different variables.
High-Dimensional Causal Inference: In scenarios where the number of measured variables is significantly larger than the sample size, it becomes crucial to employ methods that can efficiently infer causality.
Examples for Investors:
The Graphical Lasso and its variants play a pivotal role in understanding the complex web of relationships in high-dimensional datasets, which can be instrumental for investors aiming to optimize their strategies and manage risks.
Portfolio Optimization: Investors can use the Graphical Lasso to deduce the relationships between different assets in a portfolio. By understanding these relationships, they can make better decisions about which assets to include/exclude, thereby optimizing the portfolio's performance.
Risk Management: By understanding causal relationships between various economic indicators, market sectors, and individual assets, investors can predict potential risks and take measures to mitigate them.
Fund Analysis: For investors looking into mutual funds or hedge funds, understanding the causal relationships between different investment strategies and their returns can be crucial. This can be achieved using methods like the Multi-task Attributed Graphical Lasso.
Other Advanced Tools:
Tractable Probabilistic Circuits for Causal Inference: Recent developments in probabilistic models, especially tractable probabilistic circuits, have shown promise in efficiently computing properties such as marginal probabilities, entropies, expectations, and other related queries. These probabilistic circuit models can be effectively learned from data, making them a suitable foundation for causal inference algorithms.
Neural Networks and Spurious Correlations: Neural networks, especially when trained on large datasets, can inadvertently pick up on spurious correlations present in the dataset. These spurious correlations can impact the network's ability to generalize well to unseen data or under distribution shifts. Training methodologies are being developed to make neural networks robust to known spurious correlations. Interestingly, some counter-intuitive observations have emerged regarding the effect of model size and training data on these correlations.
Restricted Boltzmann Machines (RBMs) in Graphical Models: Graphical models are powerful tools for describing high-dimensional distributions in terms of their dependence structure. While algorithms for learning undirected graphical models have been developed, the presence of latent variables (unobserved) introduces challenges. RBMs are popular models with broad applications. A straightforward greedy algorithm has been proposed for learning ferromagnetic RBMs. This algorithm's analysis utilizes tools from mathematical physics, specifically those developed to demonstrate the concavity of magnetization.
Learning Gaussian Graphical Models (GGMs): Gaussian Graphical Models are extensively employed in machine learning and other scientific domains to model statistical relationships between observed variables. These models are especially useful when the number of observed samples is much smaller than the dimension. Algorithms like Graphical Lasso and CLIME can provably recover the graph structure with a logarithmic number of samples. However, they often assume conditions related to the precision matrix being well-conditioned. New algorithms have been introduced that can learn specific types of GGMs with a logarithmic number of samples without making such assumptions.
Challenges and Limitations
Data Quality: Even the most sophisticated tools are only as good as the data fed into them. Inaccurate, outdated, or biased data can lead to misleading results. Decisions based on poor-quality data can lead to financial losses and missed investment opportunities.
Complexity of Interpretation: Advanced tools often produce complex models that require expertise to interpret correctly. Misinterpretations can lead to misguided strategies, causing investors to potentially make suboptimal choices.
Over-reliance on Automation: There's a temptation to fully trust automated insights without questioning or cross-referencing them. Blindly following algorithmic recommendations without human judgment can lead to overlooking nuances or external factors the model might have missed.
Scalability Issues: Some advanced tools, especially when dealing with vast datasets, can face scalability challenges, taking longer times to process or requiring more computational power. Delays in analysis can result in missed timely investment opportunities or increased operational costs.
Potential for Overfitting: Some models, especially complex ones, might fit the training data too closely, capturing noise rather than the underlying pattern. Overfitted models might perform poorly on new data, leading to unreliable predictions for real-world scenarios.
Lack of Transparency: Many advanced tools, especially neural networks, are often dubbed as "black boxes" because their decision-making processes are not easily interpretable. Without clear insight into how decisions are made, it can be challenging to trust or justify the results, especially in regulatory or high-stakes environments.
The field of causal inference, especially when integrated with graphical models like Graphical Lasso, is rich with complexities and nuances. Algorithmic advancements and computational tools like tractable probabilistic circuits, neural networks, RBMs, and GGMs are shaping the way we understand and infer causality in high-dimensional datasets. For investors and researchers, understanding these methods can provide a robust foundation for deciphering intricate relationships in data and making informed decisions.
Comentarios