Active inference is a theoretical framework in the field of artificial intelligence (AI) that seeks to explain how intelligent agents can efficiently interact with their environment. It draws inspiration from the principles of Bayesian inference, predictive coding in neuroscience, and cybernetics. Active inference proposes that intelligent agents aim to minimize uncertainty or surprise in their interactions with the world by continually updating their beliefs and taking actions to gather more information.
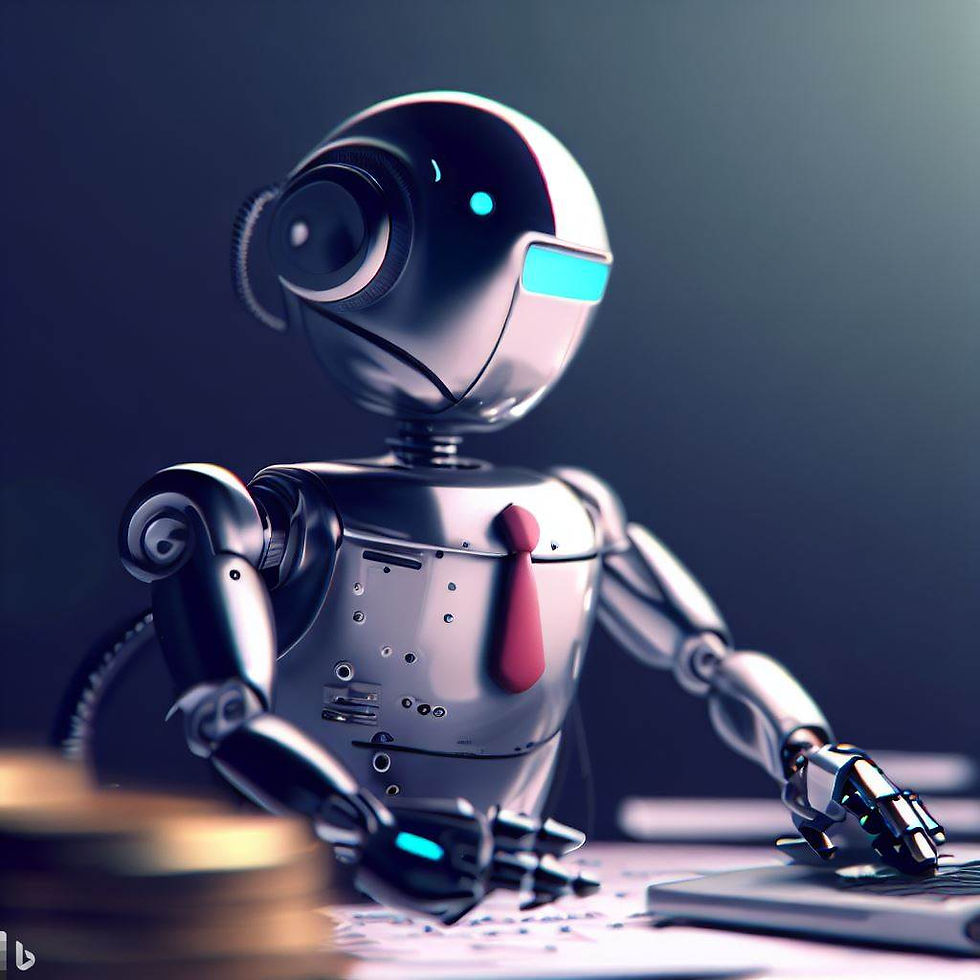
The core idea behind active inference is that agents have internal models of the world that capture their beliefs about the underlying causes and dynamics of the environment. These models are used to make predictions about sensory inputs based on the agent's current beliefs. When there is a mismatch between the predicted and actual sensory inputs, this is considered as surprise or prediction error.
To minimize surprise, active inference suggests that agents should take actions that maximize the evidence for their current beliefs or reduce uncertainty. These actions are chosen based on the agent's internal model, which includes both prior beliefs about the environment and the agent's own capabilities. The agent's actions are intended to gather new information that will help reduce uncertainty and bring the predicted sensory inputs closer to the actual ones.
Active inference can be seen as a form of embodied cognition, where the agent actively engages with the environment to reduce uncertainty and improve its internal model. This is in contrast to passive inference, where the agent simply observes the environment without actively influencing it.
The active inference framework provides a theoretical basis for understanding how intelligent agents can learn, plan, and act in a goal-directed manner. By continually updating their beliefs and selecting actions to minimize surprise, agents can adapt to changes in the environment, make accurate predictions, and achieve their goals more effectively. It is important to note that active inference is still a developing field of research, and its practical application in AI systems is an ongoing area of exploration.
Active inference and large language models (LLMs) like the GPT series from OpenAI are fundamentally different in their approach and application.
Active inference is a theoretical framework that focuses on how intelligent agents interact with their environment to reduce uncertainty and minimize surprise. It is a general framework that can be applied to various domains beyond language processing, such as robotics, perception, and decision-making. Active inference emphasizes the agent's active engagement with the environment, where actions are taken to gather new information and update internal beliefs. On the other hand, LLMs like GPT are language models that are designed specifically for natural language processing tasks, such as text generation, translation, summarization, and question answering. These models are trained on vast amounts of text data and learn to predict the likelihood of specific words or phrases given a context. LLMs do not actively interact with the environment or take actions to reduce uncertainty; instead, they generate text based on statistical patterns learned during training.
LLMs are primarily focused on language understanding and generation, whereas active inference is a broader framework that encompasses decision-making, perception, and action. LLMs excel at processing and generating human-like text, while active inference provides a theoretical framework for intelligent agents to actively engage with the world. In summary, active inference is a theoretical framework for intelligent agent behavior, emphasizing the active interaction with the environment to minimize surprise, while LLMs like GPT are language models specifically designed for natural language processing tasks.
The Role of Active Inference in Financial Contexts
Recent advancements in artificial intelligence have led to the development of powerful language models, such as the GPT series from OpenAI. These large language models (LLMs) have revolutionized natural language processing tasks, demonstrating impressive capabilities in text generation, translation, and more. However, exploring the potential of active inference in the realm of finance presents an exciting opportunity to go beyond language processing and delve into intelligent decision-making and adaptive behavior.
Understanding LLMs and their Limitations: LLMs like GPT are trained on vast amounts of text data, learning to predict the likelihood of specific words or phrases given a context. They excel in generating coherent and contextually appropriate text, making them valuable for various language-related applications. However, LLMs have inherent limitations, as they lack the ability to actively engage with the environment, update their beliefs, and make adaptive decisions based on real-time data. This limitation becomes apparent when dealing with dynamic and uncertain domains like finance.
Introducing Active Inference: Active inference provides a theoretical framework for intelligent agents to actively interact with their environment, update their beliefs, and minimize surprise or uncertainty. Applying active inference to finance allows agents to make more informed and adaptive decisions, enhancing their ability to navigate complex market dynamics. Active inference involves three main components: generative models, inference, and action.
Generative Models in Finance: Generative models capture an agent's beliefs about the underlying causes and dynamics of the financial environment. These models encompass factors like market trends, risk factors, investor behavior, and economic indicators. By incorporating these factors, generative models enable agents to simulate and make predictions about the financial landscape.
Inference in Finance: Inference refers to the process of updating beliefs based on incoming data. In the context of finance, agents utilizing active inference continuously gather real-time financial data, such as stock prices, economic reports, news sentiment, and investor sentiment. By comparing this data to their generative models, agents can identify discrepancies and update their beliefs accordingly. This process allows for adaptive decision-making in response to changing market conditions.
Action in Finance: Action represents the agent's response to the updated beliefs. In the finance domain, agents employing active inference may take actions such as buying or selling securities, adjusting investment portfolios, or managing risk exposure. These actions are aimed at reducing uncertainty, maximizing expected returns, and aligning the agent's position with the updated beliefs about the financial landscape.
Example 1: Portfolio Management: Consider an investment firm utilizing active inference in portfolio management. The firm's generative model captures market trends, asset correlations, and risk factors. Real-time financial data, including stock prices, economic indicators, and news sentiment, are continuously collected. By comparing the incoming data to the generative model, the firm identifies opportunities and risks. Based on these insights, the firm adjusts its investment allocations, rebalancing the portfolio to optimize returns and manage risk effectively.
Example 2: Trading Strategies: Active inference can also enhance trading strategies in finance. A trading firm employing active inference utilizes generative models that incorporate historical price data, technical indicators, and market volatility. By continuously comparing real-time market data to their models, the firm detects patterns and anomalies, updating their beliefs about market conditions. Based on these updates, the firm executes trades, adapting their strategies to capitalize on market opportunities or mitigate risks.
Implications and Benefits of Active Inference in Finance: Integrating active inference into financial systems offers several key advantages. Firstly, it enables real-time adaptive decision-making, allowing agents to respond rapidly to changing market conditions. Secondly, active inference provides a means to handle uncertainty and manage risk more effectively. By continually updating beliefs and taking actions to minimize surprise, agents can navigate volatile market environments with greater resilience. Furthermore, active inference promotes a more holistic approach to finance, considering not only numerical data but also qualitative factors such as investor sentiment and market trends. Active inference in finance also opens doors for personalized financial services. By tailoring generative models and actions to individual investors' preferences, risk tolerance, and financial goals, active inference-based systems can provide more customized investment advice and portfolio management strategies.
Moreover, active inference can aid in detecting market anomalies and predicting market trends. By identifying discrepancies between predicted and observed data, agents can uncover potential market inefficiencies or emerging opportunities that might be missed by traditional models. This can lead to improved investment strategies and potentially higher returns. However, it is crucial to acknowledge that active inference in finance is still an emerging area of research and application. Challenges lie in accurately modeling the complex dynamics of financial markets and effectively incorporating vast amounts of real-time data. Additionally, ethical considerations and regulatory frameworks need to be taken into account to ensure the responsible use of active inference in finance.
Active inference provides a promising framework to extend the capabilities of language models like GPT series into the realm of intelligent decision-making in finance. By combining generative models, inference, and action, active inference enables agents to actively engage with the financial environment, update beliefs based on real-time data, and make adaptive decisions to minimize uncertainty and maximize returns. The implications of active inference in finance include personalized financial services, enhanced risk management, anomaly detection, and improved investment strategies. However, further research and development are needed to address challenges and ensure the responsible implementation of active inference in the financial domain.
Interesting fact: While Active Inference itself is a concept from the field of neuroscience and cognitive science, its application in the realm of finance is quite fascinating. It provides a unique framework for understanding how humans and artificial systems can make decisions under uncertainty, which is a constant feature of financial markets. What's interesting is that Active Inference applies principles of Bayesian statistics and machine learning to model how entities can predict market trends, optimize investment strategies, and manage financial risks, all based on limited, uncertain information. It's a significant demonstration of the interdisciplinary nature of modern finance.
Comentarios