Causal inference is a concept that plays a critical role in the world of investing. It involves determining the cause-and-effect relationship between different variables, and it's an essential tool for making informed investment decisions. However, the process of causal inference isn't always straightforward, and one of the main challenges that investors face in this regard is the issue of confounding variables.
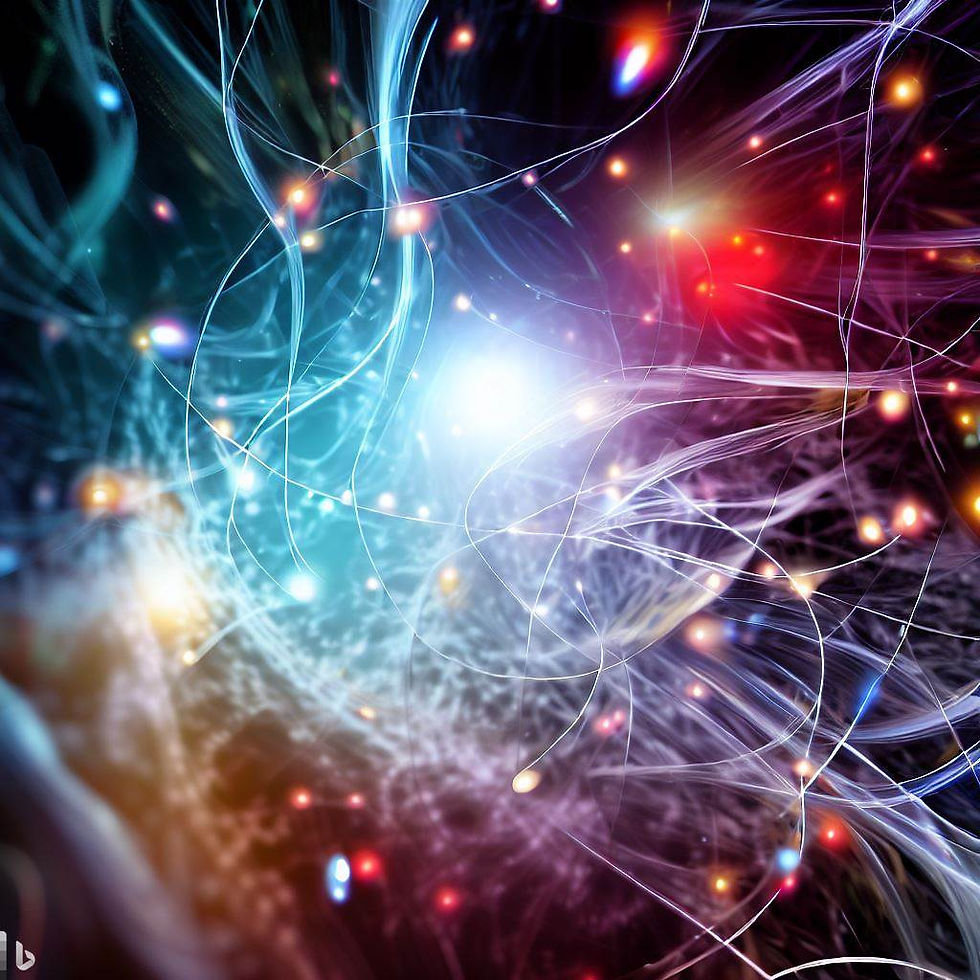
What are Confounding Variables?
In statistics, a confounding variable (also known as a confounder, confounding factor, or lurking variable) is an extraneous variable in a statistical model that correlates (positively or negatively) with both the dependent variable and the independent variable. A confounding variable can cause or prevent the outcome of interest, be associated with the factor under investigation, and hide the true association or falsely demonstrate an association where one does not exist. In the context of causal inference, confounding variables are factors that the analyst didn't account for. They can create the illusion that a particular cause-and-effect relationship exists when it doesn't, or they can hide a relationship that's actually there. For example, suppose an investor is trying to determine whether a company's advertising spend (independent variable) has a direct impact on its sales revenue (dependent variable). A confounding variable in this case could be the state of the economy. If the economy is doing well, people might be more willing to spend money, which could increase a company's sales. This could create the illusion that the advertising spend is driving sales, when in reality, it's the state of the economy.
Why are Confounding Variables Important in Investing?
Confounding variables can significantly impact the results of your analysis and lead to incorrect conclusions, resulting in potentially costly investment decisions. For example, if you incorrectly conclude that a particular factor is driving a company's growth, you might invest heavily in that company expecting that growth to continue. If, in reality, the growth was due to a confounding variable that you didn't account for, you could end up losing money when that variable changes. Understanding and controlling for confounding variables can lead to more accurate causal inferences and better investment decisions. It allows investors to separate the true drivers of a phenomenon from the noise, focusing their attention and resources on the factors that truly matter.
How to Deal with Confounding Variables in Investing
Identify Potential Confounders: The first step in dealing with confounding variables is to identify them. This can be done through a thorough understanding of the domain, using logical reasoning, or utilizing statistical techniques such as correlation matrices.
Collect Data on Confounding Variables: Once you've identified potential confounders, you need to collect data on these variables. This might involve additional data collection efforts, but it's an essential step in controlling for confounding variables.
Use Statistical Methods to Control for Confounding Variables: There are several statistical methods you can use to control for confounding variables, including stratification, matching, regression adjustment, and propensity score methods. These methods essentially allow you to isolate the effect of the variable you're interested in from the effects of the confounding variables.
Use Experimental Design: If possible, using experimental design can help to control for confounding variables. Randomized controlled trials (RCTs) are the gold standard in this regard, as they randomly assign subjects to different groups to ensure that the groups are similar except for the variable being studied. However, RCTs may not always be feasible or ethical in many investment contexts.
Examples of Confounding Variables in Investing
Let's consider a few examples to illustrate the concept of confounding variables in investing.
Example 1: Technology Investments and Economic Conditions: Suppose an investor is examining the relationship between investing in technology stocks and overall portfolio performance. They might find that their portfolio performs better when they invest more in technology stocks. However, this could be confounded by overall economic conditions. During periods of economic growth, both technology stocks and the overall portfolio might perform well, creating a confounding relationship.
Example 2: CEO Changes and Stock Prices: An investor might be interested in the effect of CEO changes on stock prices. They might observe that when a company changes its CEO, the stock price tends to increase. However, this could be confounded by the reasons for the CEO change. If the CEO was changed due to poor performance, then any change in strategy or improvement in performance might lead to an increase in stock price, not the CEO change itself.
AI and Confounding Variables in Causal Inference
Artificial Intelligence (AI) has the potential to significantly aid in the process of identifying and controlling for confounding variables in causal inference, leading to more accurate and informed investment decisions. Here's how:
Identification of Confounding Variables: AI algorithms, especially those involving machine learning, can process large datasets and identify patterns and relationships that would be difficult, if not impossible, for a human to discern. By analyzing these patterns, AI can help identify potential confounding variables that might be affecting the relationship between the independent and dependent variables. For instance, advanced AI algorithms like random forests and neural networks can handle high-dimensional data and automatically capture interactions between variables, potentially highlighting confounders.
Control of Confounding Variables: Once potential confounding variables have been identified, AI can also assist in controlling for these variables. This is typically done through a process called 'feature engineering', in which new variables are created to capture the confounding effect. For example, if an AI model identifies that both economic conditions and technology investment are important predictors of portfolio performance, it might create a new feature that captures the interaction between these two variables. This feature would then be included in the model, allowing the AI to isolate the impact of technology investment on portfolio performance.
Causal Inference: AI can go a step further with causal inference by using advanced techniques such as Causal Bayesian Networks and Directed Acyclic Graphs (DAGs). These methods are capable of capturing the causal relationships between variables, including the impact of potential confounders.
Predictive Modeling: AI models can forecast the impact of changes in independent variables (including confounding variables) on the dependent variable, which is particularly valuable for investors. For example, an AI model might predict how changes in a company's advertising spend, as well as changes in the economy, will impact that company's sales. This predictive capability can help investors make more informed decisions about where to invest.
Automation and Speed: AI can process and analyze data much faster than humans can, allowing for real-time insights and quicker decision-making. This is particularly valuable in the fast-paced world of investing, where being able to quickly adjust to new information can make the difference between profit and loss.
Confounding variables can significantly impact the conclusions drawn from causal inference in investing. By understanding, identifying, and controlling for confounding variables, investors can make more informed and accurate investment decisions. AI offers powerful tools for identifying and controlling for confounding variables in causal inference. By leveraging these tools, investors can gain a deeper understanding of the factors driving their investment returns and make more informed investment decisions. However, like all tools, AI is not without its limitations and should be used in conjunction with human expertise and judgement.
Comentarios