Causal inference is a core principle of scientific reasoning and is crucial in many fields, including finance and investing. While the phrase "correlation does not imply causation" is often repeated, the practical implications and application of this in finance are perhaps not as well understood. This article aims to shed light on the topic of causal inference in finance, including what it is, why it's important, and how it can be applied, with practical examples.
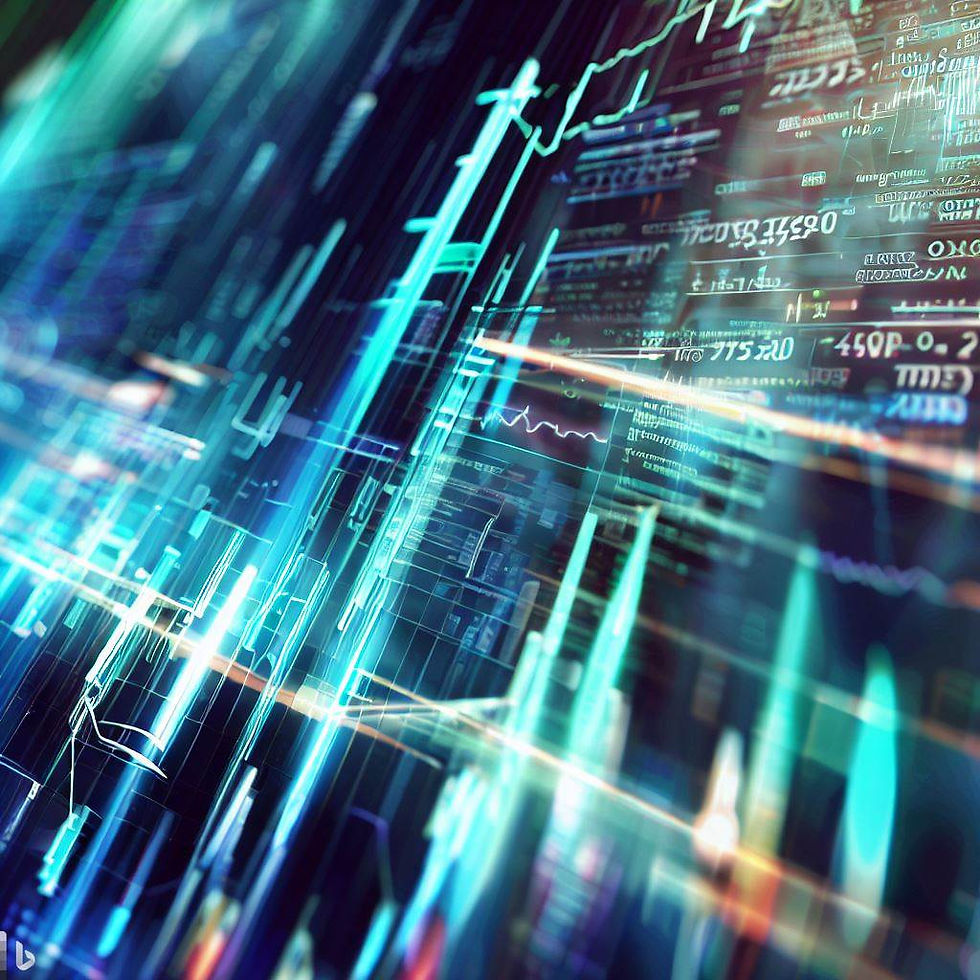
What is Causal Inference?
In the simplest terms, causal inference refers to the process of determining whether one event or condition (cause) directly results in another event or condition (effect). This is different from correlation, which only tells us that two variables move together, without necessarily implying a cause-and-effect relationship. Causal inference attempts to answer the question "What would happen if...?" It seeks to identify not just relationships, but the direction, magnitude, and nature of cause-and-effect relationships. This is often more challenging because it requires us to consider hypothetical scenarios that didn't actually occur.
Why is Causal Inference Important in Finance and Investing?
Understanding cause-and-effect relationships is crucial in finance and investing for several reasons:
Making Predictions: If we know that A causes B, we can predict B when A occurs. This is crucial for investment strategies, which often rely on predicting future prices or economic conditions.
Understanding Mechanisms: Causal relationships help us understand why things happen, not just that they happen. This deeper understanding can help investors make more informed decisions.
Policy and Regulation: Understanding the causal impact of policy decisions or regulatory changes can help investors anticipate how these factors might affect their investments.
Causal Inference Methods in Finance
There are several methods and approaches used in finance to infer causality. Here are some of the most common ones:
Randomized Controlled Trials (RCTs): RCTs are the gold standard for causal inference in many fields, including medicine and economics. In a financial context, an RCT might involve randomly assigning different investment strategies to different groups and comparing the outcomes. However, RCTs are often not feasible in finance due to ethical or logistical constraints.
Natural Experiments: When an RCT isn't possible, a natural experiment can be a good alternative. This involves finding a situation where the variable of interest is assigned randomly or as if it were random. For example, changes in regulations or policy often affect only certain companies or sectors, creating a natural experiment.
Instrumental Variables (IVs): An IV is a variable that is correlated with the independent variable but not with the error term. It can be used as a proxy to infer causality when direct measurement is not possible. In finance, an example might be using rainfall as an instrumental variable to study the impact of agricultural output on stock prices.
Difference-in-Differences (DiD): This method compares the change in outcomes over time between a treatment group (affected by an event or policy) and a control group (not affected). It's commonly used in finance to study the impact of changes in laws or regulations.
Example: The Influence of Monetary Policy on Equity Markets:
Monetary policy, typically embodied by the adjustment of interest rates, serves as a significant tool for central banks to manage inflation and stimulate economic progress. The question that often arises for investors is: Does a shift in interest rates directly lead to changes in stock prices?
While a simple analysis might indicate a correlation between interest rates and stock prices, it falls short of clarifying whether changes in interest rates are the driver behind the movements in stock prices, or if a third variable (such as economic growth) is the underlying impetus affecting both.
The technique of Instrumental Variable (IV) analysis can provide a more nuanced perspective, allowing us to infer causality between monetary policy and stock prices. The crux of this method lies in identifying an appropriate instrument—a variable that shares a correlation with monetary policy (our independent variable), but is not correlated with the error terms.
Consider a variable like the annual number of policy meetings held by the central bank, which is influenced by the central bank policy but doesn't directly impact stock prices. This variable could be a potential instrument. The reasoning is that the number of meetings could indirectly influence stock prices by impacting the policy, yet it has no direct link to stock prices.
To leverage this instrument, we would employ a method known as two-stage least squares regression (2SLS), commonly used in IV analysis. In the first stage, we predict the values of the monetary policy variable (for instance, interest rates) based on our instrument (number of policy meetings). In the second stage, these predicted values are used to estimate their impact on stock prices. This approach allows us to isolate the causal effect of monetary policy on stock prices, provided our instrument holds validity—that is, it meets the requirements of relevance and exclusion restriction conditions.
Example: The Effect of Leadership Transition on Corporate Performance:
In the world of finance, a frequently asked question concerns the impact of leadership transitions—specifically, the appointment of a new CEO—on a company's performance. One method to investigate this is through the difference-in-differences (DiD) approach.
Visualize a scenario where we possess data on a set of companies that experienced a CEO transition (our treatment group), along with data on a comparable set of companies that did not undergo such a change (our control group). We would then observe their performance indicators, such as stock returns, both before and after the CEO transition event in the treatment group.
The initial step involves computing the difference in performance for each group, pre and post the CEO transition. Following this, we compare the differences between the two groups. If we observe a significantly larger change in performance in the treatment group—the companies that underwent a CEO change—compared to the control group, it would suggest a causal relationship.
It is important to note that these examples provide a simplified snapshot of the complexities and intricacies involved in causal inference, which often involves careful study design, rigorous statistical analysis, and thoughtful interpretation.
Applying Causal Inference in AI for Finance and Investing
The integration of Artificial Intelligence (AI) into the finance and investment sectors has opened new avenues for applying causal inference. Machine learning models, with their ability to analyze vast amounts of data and discern patterns, can significantly enhance our ability to infer causality. However, as they are typically correlation-based, it's necessary to develop and adapt these models to focus on causal relationships.
Causal Machine Learning (Causal ML) is one such development that seeks to integrate causal inference principles into machine learning techniques. It aims to move beyond mere predictive modelling to uncover the underlying causal relationships among variables, thus allowing for more robust and reliable decision making in finance and investing.
Example: AI and Causal Inference for Loan Approval:
A traditional AI model for loan approval would utilize machine learning to analyze vast amounts of data and identify patterns or correlations that indicate the likelihood of loan repayment. However, this approach, while effective at predicting outcomes based on historical data, may not necessarily uncover the causal relationships that drive these outcomes. For instance, it may find a correlation between a borrower's postcode and their likelihood of repaying a loan, but it does not necessarily mean that changing someone's postcode would make them more likely to repay a loan.
In contrast, a Causal ML model would seek to identify factors that, when altered, would actually change the likelihood of loan repayment. For instance, it might identify that increasing a borrower's income (a causal factor) would increase their likelihood of repaying a loan.
Example: Portfolio Management and Causal Inference:
A common issue in portfolio management is understanding which factors truly drive the performance of a given portfolio. Traditional AI models might identify correlations between various economic indicators and portfolio performance. However, these correlations might not necessarily represent causal relationships.
Applying Causal ML can help portfolio managers identify true causal relationships, allowing them to make more informed decisions. For instance, if a Causal ML model identifies a specific company's earnings as a causal factor in portfolio performance, a portfolio manager might focus more on those earnings reports when making investment decisions.
Potential Limitations and Ethical Considerations
Despite its promise, the application of Causal ML in finance and investing also comes with challenges and ethical considerations. For instance, the quality of causal inference is heavily dependent on the quality of the data and the assumptions made in the model. False assumptions or biases in the data can lead to spurious causal relationships, leading to potentially harmful decisions.
Furthermore, while AI and Causal ML can provide valuable insights, they should not replace human judgment. It's crucial for financial professionals to critically interpret and validate the insights generated by these models. Ethically, it's also essential to ensure that AI models do not inadvertently perpetuate or exacerbate existing biases or inequalities.
While causal inference presents challenges, it also offers opportunities for more robust and reliable decision-making in finance and investing. By integrating causal inference principles into AI models, we can move beyond identifying correlations to uncovering the causal relationships that truly drive financial outcomes. However, it's crucial to approach these techniques with caution, carefully considering the quality of the data, the assumptions made in the models, and the ethical implications of their use.
Interesting fact: An interesting historical example of causal inference in finance is the study of the Black Monday stock market crash in 1987. The largest one-day stock market drop in history, Black Monday saw the Dow Jones Industrial Average fall 22.6% in a single day. In the aftermath of the crash, economists and financial analysts sought to understand its causes. While many factors were correlated with the crash, including computerized trading and international disputes, it was challenging to identify which of these were causes and which were merely coincidental correlations. Economists used various causal inference techniques to isolate the impacts of different factors. Through these studies, they found that portfolio insurance, a financial instrument that was supposed to protect investors from market downturns, actually played a causal role in exacerbating the crash. This finding had a significant impact on the future regulation of financial markets and highlighted the importance of causal inference in understanding financial events.
Comments