Black Swan events, a term coined by Nassim Nicholas Taleb, are events that are unpredictable, have a severe impact, and are rationalized only in hindsight. The economic and financial markets have experienced several such events, like the dot-com bubble, the 2008 financial crisis, and the 2020 COVID-19 pandemic. In this article, we delve into Black Swan events and explore the evolving role of artificial intelligence (AI) in predicting, managing, and mitigating these phenomena.
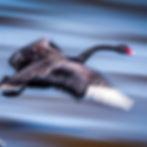
Understanding Black Swan Events
Black Swan events are rare, unforeseen, and consequential incidents that disrupt normal operations and can trigger widespread systemic effects. A couple of such events that have shaped the financial markets include:
The Dot-Com Bubble (2000): This was a speculative bubble resulting from excessive speculation in Internet-related companies during the late 1990s. When the bubble burst in 2000, many investors lost substantial sums of money, and several online businesses ceased to exist. This event was unforeseen, had a high impact, and is now rationalized as an outcome of uncontrolled speculation and valuation.
The Global Financial Crisis (2008): The 2008 crisis was triggered by the bursting of the United States housing bubble. The ripple effects were felt worldwide, leading to long-term unemployment, downturns in stock markets, and collapsed businesses. Again, the event's arrival and scale were unpredictable, but its aftermath made evident the severe flaws in global financial systems.
The Role of AI in Financial Markets
AI has become an indispensable tool in financial markets. It assists in high-frequency trading, predictive analytics, portfolio management, fraud detection, and risk management. AI algorithms are designed to learn from historical data, identify patterns, and make predictions or decisions without human intervention. For instance, AI-driven high-frequency trading has revolutionized financial markets by enabling trades within fractions of a second, something human traders cannot achieve. Predictive analytics algorithms can process vast amounts of financial data to forecast market trends. However, as impressive as these capabilities are, AI's power in predicting or mitigating Black Swan events is not straightforward.
AI and Black Swan Events
The difficulty lies in the very nature of Black Swan events. Since they are defined as unpredictable and beyond the realm of regular expectations, it becomes inherently challenging for AI, which relies on historical data and established patterns, to anticipate them. Black Swan events do not follow past trends, making them elusive to AI systems trained on historical data. Here's why:
Limitation of Historical Data: AI systems learn from past data. They find patterns and make predictions based on these patterns. However, Black Swan events, by definition, do not conform to past trends or patterns. They are outliers with extreme impacts.
Overfitting: Overfitting is a common problem in machine learning where a model performs well on the training data but fails to generalize well to unseen data. If an AI model is too adapted to past data, it might fail to anticipate significant deviations like Black Swan events.
Lack of Contextual Understanding: AI lacks the human ability to understand context. While it can crunch numbers and identify patterns faster than humans, it may not comprehend complex political, social, or environmental factors that could trigger a Black Swan event.
However, this does not mean AI is entirely powerless in the face of Black Swan events.
AI's Role in Managing and Mitifying Black Swan Events
Despite its inability to predict Black Swan events, AI can play a significant role in managing and mitigating the impacts of these events. Here's how:
Real-time Data Processing: AI's ability to process large amounts of data in real-time can help identify the onset of a Black Swan event faster than traditional methods, enabling quicker responses.
Risk Management: Machine learning models can identify vulnerabilities in a system or a portfolio that could exacerbate the impact of a Black Swan event. By identifying these vulnerabilities early, organizations can take measures to mitigate potential risks.
Crisis Management: AI can assist in managing a crisis by automating certain processes, thus allowing human decision-makers to focus on strategic considerations.
Post-event Analysis: After a Black Swan event, AI can be used to analyze the event's impacts, understand its dynamics, and prepare better for similar events in the future. This analysis can provide valuable insights for strengthening resilience in financial markets.
While Black Swan events pose significant challenges to the financial markets, AI offers innovative ways to manage and mitigate these events' impacts. As we continue to navigate a world increasingly influenced by unforeseen global events, leveraging AI's capabilities will be crucial. However, it's important to remember that AI is not a silver bullet, and its use should be complemented by sound human judgment and robust risk management frameworks. With a balanced approach, investors can better navigate the unpredictable waters of the financial market, even in the face of Black Swans.