Beyond Words: How Animal-Inspired Non-Linguistic Intelligence Can Reshape AI Development
- Aki Kakko
- 4 minutes ago
- 5 min read
The current landscape of Artificial Intelligence is still heavily dominated by breakthroughs in Large Language Models (LLMs). Systems like GPT-series, Claude, and Llama showcase remarkable abilities in understanding, generating, and manipulating human language. While undeniably powerful, this focus risks creating a skewed perception of intelligence, anchoring it primarily to linguistic prowess. However, the natural world offers a staggering diversity of intelligence that operates entirely outside the realm of human language. By studying and drawing inspiration from the non-linguistic intelligence of animals, we can unlock new pathways for AI development, leading to systems that are potentially more robust, adaptable, efficient, and grounded in the physical world.
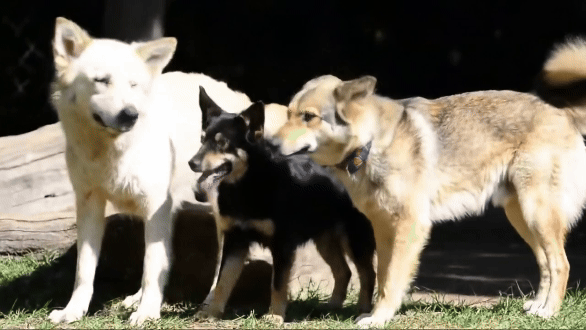
What is Animal Non-Linguistic Intelligence?
Animal intelligence isn't a monolithic concept. It encompasses a vast array of cognitive abilities fine-tuned by millions of years of evolution to solve specific survival and reproductive challenges within complex, dynamic environments. Key aspects relevant to AI include:
Sophisticated Perception & Sensory Integration: Animals process information from multiple senses (sight, sound, smell, touch, echolocation, electroreception, etc.) simultaneously, integrating them into a coherent model of their surroundings to detect prey, avoid predators, and navigate.
Spatial Reasoning & Navigation: From ants finding the shortest path back to the nest to birds migrating thousands of miles, animals exhibit exceptional spatial awareness and navigational skills, often using internal maps, celestial cues, magnetic fields, and landmarks.
Motor Control & Embodied Cognition: Animals display incredible dexterity, agility, and coordination. Their intelligence is deeply intertwined with their physical bodies and how they interact with the environment (embodiment). Think of an octopus manipulating objects with its arms or a cheetah executing a high-speed chase.
Adaptive Problem Solving: Animals encounter novel situations and devise solutions without explicit instructions. This includes tool use (crows, primates), escaping traps, finding hidden food, and adapting hunting strategies.
Social Cognition & Communication (Non-Verbal): Many animals live in complex social structures, requiring them to understand social cues, hierarchies, intentions, and coordinate group behaviour (e.g., pack hunting, flocking, colony organization) through non-linguistic signals like posture, scent, calls, and coordinated movement.
Learning & Memory: Animals learn from experience, associate stimuli with outcomes (classical and operant conditioning), learn by observation, and retain information crucial for survival.
While LLMs excel at text-based tasks, they often struggle with:
Grounding: Connecting language symbols to real-world concepts, physics, and causality can be weak. They lack true "understanding" in the human sense.
Common Sense Reasoning: Especially regarding the physical world and intuitive physics.
Robustness & Adaptability: They can be brittle, failing unexpectedly when faced with inputs slightly outside their training distribution or requiring real-time adaptation to dynamic physical environments.
Embodiment & Physical Interaction: Most LLMs lack a physical body and struggle with tasks requiring direct, nuanced interaction with the physical world.
Data & Energy Efficiency: Training large models requires massive datasets and computational resources, whereas animals often learn efficiently from limited interactions.
How Animal-Inspired Non-Linguistic AI Can Benefit Development
Drawing inspiration from animal intelligence offers solutions and new perspectives to overcome these limitations and enrich AI development in several key areas:
Enhanced Robustness and Adaptability:
Animal Inspiration: Animals thrive in unpredictable, noisy environments. Their sensory processing and decision-making are inherently robust against partial information and changing conditions. Social insects like ants demonstrate decentralized, resilient colony behaviour.
AI Benefit: Designing AI systems (especially for robotics, autonomous vehicles, and IoT) that are less brittle and can gracefully handle unexpected events, sensor noise, or failures. Developing algorithms inspired by swarm intelligence for robust, decentralized control systems.
Superior Spatial Reasoning and Navigation:
Animal Inspiration: The navigational prowess of bees (using polarized light, sun compass, optic flow), bats (echolocation for 3D mapping), and migrating birds offers blueprints for efficient spatial cognition.
AI Benefit: Developing more capable AI for autonomous navigation in complex, unknown environments (drones, self-driving cars, underwater exploration). This includes better simultaneous localization and mapping (SLAM), path planning, and sensor fusion techniques inspired by biological solutions.
Grounded Intelligence through Embodiment and Sensorimotor Skills:
Animal Inspiration: Animal intelligence is inseparable from their bodies and interaction with the world. An octopus's intelligence is distributed, with arms capable of independent processing. Insect flight control is a marvel of real-time sensorimotor integration.
AI Benefit: Fostering the development of truly "embodied AI" where learning and reasoning are intrinsically linked to physical interaction. This leads to robots with better dexterity, coordination, and an intuitive grasp of physics learned through interaction, rather than just programmed rules or simulated data. Inspiration from animal locomotion can lead to more agile and efficient robots.
Efficient Learning and Resourceful Problem Solving:
Animal Inspiration: Animals often learn crucial survival skills quickly and efficiently, sometimes through trial-and-error, observation, or innate heuristics. Crows figuring out complex multi-step puzzles to get food demonstrate non-linguistic problem-solving.
AI Benefit: Developing AI algorithms that require less data, learn faster, and are more energy-efficient. Exploring reinforcement learning, curiosity-driven exploration, and developmental approaches inspired by how young animals learn. Designing AI capable of creative, non-symbolic problem-solving for physical tasks.
Richer Human-AI Interaction & Social Robotics (Non-Verbal Focus):
Animal Inspiration: Animals communicate and coordinate effectively using a rich repertoire of non-verbal cues (body language, gaze, vocalizations). Understanding intention and social dynamics is crucial in pack animals or primate groups.
AI Benefit: Creating AI agents and robots that can better understand and respond to human non-verbal cues (like posture, tone of voice, facial expressions), leading to more natural and intuitive human-robot interaction. Developing AI for coordinating teams of robots inspired by animal group behaviour (flocking, schooling, pack hunting).
Novel AI Architectures and Algorithms:
Animal Inspiration: Brains of even simple insects possess highly efficient neural circuits for tasks like navigation and odour detection. Studying these structures can inspire new, low-power, specialized AI hardware and algorithms (neuromorphic computing). The distributed, non-hierarchical nature of some animal nervous systems offers alternatives to current deep learning architectures.
AI Benefit: Moving beyond standard deep learning models to explore diverse computational paradigms. This could lead to AI that is faster, more power-efficient, and better suited for specific sensory processing or control tasks, particularly on edge devices.
Challenges and the Path Forward
Translating animal intelligence into AI is not straightforward. Challenges include:
Understanding Animal Cognition: Our knowledge of how animal brains achieve these feats is still incomplete.
Level of Abstraction: Determining whether to mimic biological mechanisms directly or abstract the underlying principles.
Integration: Combining non-linguistic, embodied intelligence with symbolic reasoning and language capabilities effectively.
Complexity: Biological systems are immensely complex and evolved; replicating them is daunting.
Despite these challenges, the potential benefits are immense. The path forward requires greater interdisciplinary collaboration between AI researchers, biologists, ethologists, neuroscientists, and roboticists.
While language is a powerful hallmark of human intelligence, it is not the only form intelligence takes. The animal kingdom provides a vast library of sophisticated, non-linguistic cognitive strategies honed by evolution for survival and adaptation in the physical world. By looking beyond words and embracing inspiration from animal perception, navigation, motor control, problem-solving, and social behaviour, AI development can move towards creating systems that are not only linguistically adept but also more robust, adaptable, physically grounded, efficient, and ultimately, more holistically intelligent. This approach complements, rather than replaces, language-centric AI, promising a future where AI can engage with the complexity of the real world in ways that are currently beyond its reach.
Comments