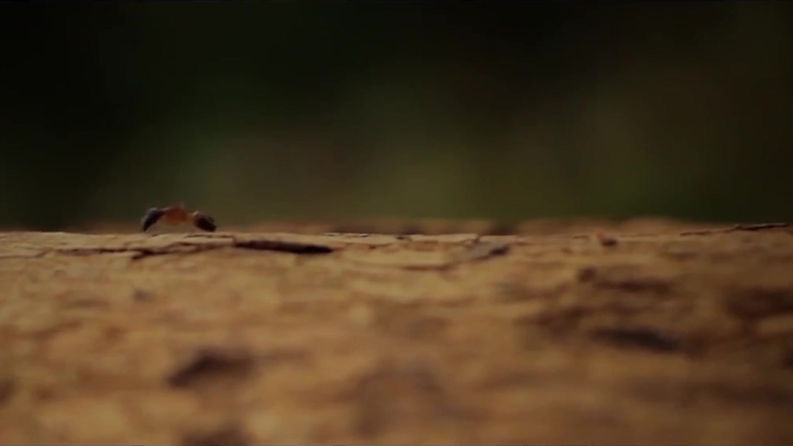
Antetic AI, inspired by ant colonies, emphasizes distributed intelligence, emergent behavior, and decentralized decision-making. Its strengths lie in scalability, robustness, adaptability, and optimization.
Antetic AI
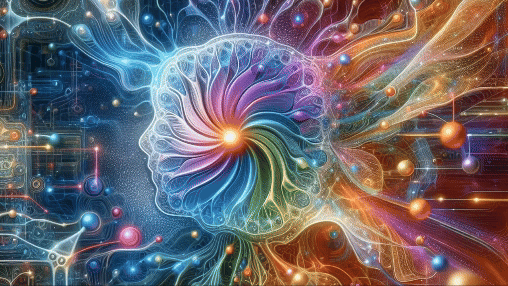
The path to Artificial General Intelligence (AGI) lies in replicating the "evolutionary kernel" of human learning – our innate predispositions and evolutionarily shaped biases. This involves endowing AI with genetically inspired core concepts (akin to an "AI genome"), implementing cultural transmission mechanisms for learning through observation, shaping curiosity with evolutionary rewards and pressures, and enabling transfer learning and analogical reasoning for adaptability. Although computationally expensive and ethically complex, mimicking natural intelligence is crucial for creating resilient, adaptable, and collaborative AI systems. Read more.
The "AntGI" hypothesis proposes that understanding the simpler, yet effective, intelligence of ant colonies offers a faster route to Artificial General Intelligence (AGI) than solely mimicking the human brain. AntGI aims to uncover fundamental algorithms and evolutionary principles by comprehensively understanding the mechanisms that allow ant colonies to solve complex problems (Dynamic Foraging and Resource Optimization; Decentralized Decision-Making and Task Allocation; Complex Nest Construction and Environmental Engineering; Social Immunity and Disease Management; Learning and Adaptation to Novel Environments; Sophisticated Communication Through Chemical Signaling). The methodology involves agent-based modeling, bio-inspired algorithm development, robotic emulation, neuroscience studies, mathematical modeling, and evolutionary computation. By focusing on the evolutionary foundations of intelligence, AntGI aims to enhance robustness, energy efficiency, and explainability in AI, while unlocking new learning paradigms and accelerating the path to AGI. It advocates for extracting fundamental principles from ant intelligence, rather than just mimicking behavior, for a more biologically plausible approach to AGI. Read more.
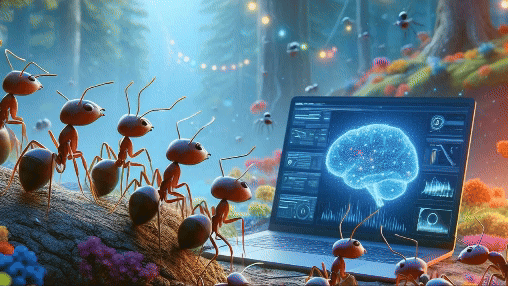
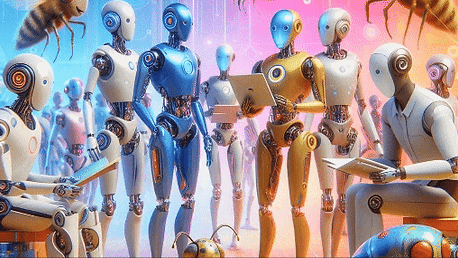_gif.gif)
Current "agentic" AIs, touted as autonomous entities, may be more like complex ant colonies ("Antetic") than truly independent agents. These AIs often use modular architectures where tasks are broken down and assigned to specialized tools (like ants with foraging duties). Techniques like Chain-of-Thought and Reflexion act as stigmergy, guiding the AI's actions, while LLMs serve as communication mechanisms ("pheromone trails") between modules. These AIs lack integrated self-awareness and often require significant upfront human priming. This raises the question: is their apparent agency an illusion created by pre-programmed systems? Recognizing this "Antetic" nature is crucial for risk mitigation, improving explainability, and focusing future research on creating genuinely autonomous AI with integrated reasoning. We need to deeply analyze the underlying architectures and carefully consider the degree to which they truly embody the principles of autonomy, proactiveness, and rationality that define genuine agency. Read more.
Antetic AI, inspired by ant colonies, emphasizes distributed intelligence, emergent behavior, and decentralized decision-making. Its strengths lie in scalability, robustness, adaptability, and optimization, but it lacks individual creativity and can face communication challenges. Agentic AI, conversely, focuses on creating individual, autonomous agents capable of independent thought, reasoning, and action. Its strengths are individual creativity, complex reasoning, and proactive behavior, but it faces complexity, scalability, and coordination issues. The future of AI may involve a convergence of these approaches, combining the strengths of both for hybrid systems. Both approaches face challenges: controlling emergent behavior in Antetic AI and achieving true autonomy and ethical behavior in Agentic AI. Read more.
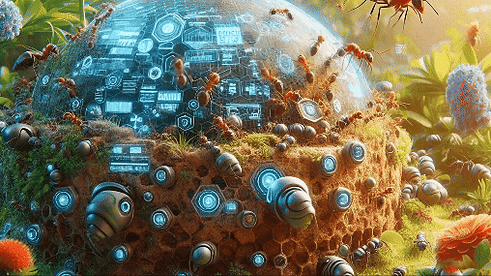_gif.gif)
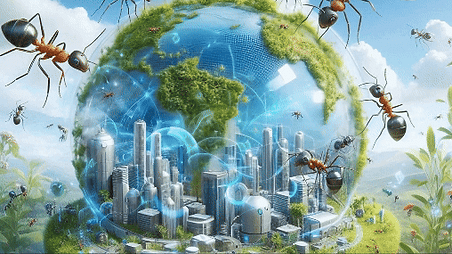_gif.gif)
Antetic AI's core principle is emergence: complex behavior arising from simple agents' interactions, making the whole more capable than the sum of its parts. Examples include optimal pathfinding via pheromone trails, dynamic task allocation responding to colony needs, complex nest construction guided by local cues, collective nest site selection through scout ant communication, and self-organized sorting/clustering. Factors influencing emergence include agent complexity, interaction rules, environmental factors, feedback mechanisms, and the number of agents. This leads to robustness, adaptability, scalability, and simplicity. Challenges include unpredictability, controlling emergent behavior, and explainability. Future research focuses on sophisticated agent models, new interaction rules, diverse applications, visualization tools, and a deeper theoretical understanding of emergence, to unlock the power of collective intelligence. Read more.
Multi-Agent Systems (MAS) often fail due to poor task specification, inter-agent misalignment, and inadequate verification. Antetic AI, inspired by ant colonies, offers a solution through decentralization, stigmergic communication, robustness, and emergent problem-solving. It addresses MAS failures by minimizing explicit task specification, reducing communication risks, and providing inherent redundancy for task verification. Specific techniques include pheromone-based task allocation, swarm robotics for collaborative construction, stigmergic information sharing, and distributed optimization algorithms. This leads to increased robustness, enhanced adaptability, improved scalability, and reduced complexity in MAS. Antetic principles can transform fragmented MAS into thriving "colonies" of intelligent agents, capable of tackling complex challenges with efficiency and resilience. Read more.
_gif.gif)
_gif.gif)
Antetic AI and Complex Systems Theory are deeply intertwined. Complex Systems Theory provides tools to understand emergence, self-organization, and feedback loops in Antetic AI, where ant behavior embodies these principles. Examples include foraging strategies, nest construction, and task allocation emerging from local interactions. Applying Complex Systems Theory aids in understanding, predicting, controlling, and optimizing Antetic AI systems, designing robust systems, and identifying key parameters. Techniques include modeling pheromone dynamics, agent-based modeling, network analysis, and sensitivity analysis. Challenges involve modeling complexity, data requirements, and computational cost. The symbiotic relationship unlocks AI that adapts, learns, and thrives in complex environments, requiring an interdisciplinary approach. Read more.
Stigmergy, a form of indirect communication where agents modify their environment to influence others, is key to Antetic AI. Unlike direct communication, it relies on agents leaving traces that act as cues. A classic example is pheromone trails in ant colonies. Key characteristics include indirect communication, environmental modification, the environment as memory, emergent coordination, scalability, and robustness. Antetic AI leverages stigmergy for pheromone-inspired path planning, stigmergic task allocation, collaborative construction with digital blocks, swarm-based data clustering, and Chain-of-Thought reasoning with a shared knowledge graph. Challenges include designing effective environmental modifications, managing complexity, balancing exploration and exploitation, and integrating individual learning. Future research will focus on more sophisticated mechanisms, individual learning, and diverse applications. Read more.
_gif.gif)
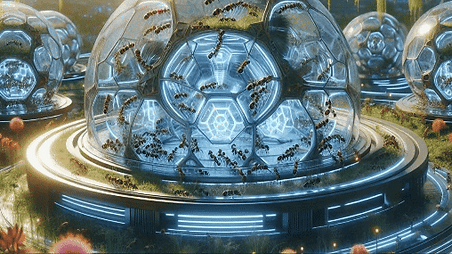_gif.gif)
Resilience is paramount in AI systems, and Antetic AI, inspired by ant colonies, offers a fault-tolerant and self-healing approach. Ant colonies achieve this through decentralized control, redundancy, adaptive communication, and self-organization. Antetic AI incorporates mechanisms like redundancy and task replication, decentralized task allocation, stigmergic communication, agent monitoring, adaptive behavior through learning, modular design, and environment-based self-repair. Examples include swarm robotics for search and rescue, distributed computing, and sensor networks. Challenges include design complexity, performance-robustness trade-offs, scalability, and verification. Future research will focus on sophisticated detection mechanisms, efficient reallocation algorithms, integrating learning, and developing theoretical frameworks. The goal is to build enduring AI systems that thrive in the face of adversity, mimicking the resilience of ant colonies. Read more.
While often seen as detrimental, noise and randomness can be powerful in Antetic AI by breaking symmetry, enhancing exploration, promoting robustness, and facilitating adaptation. Ant colonies incorporate noise through random foraging, probabilistic decision-making, and genetic variation. Antetic AI systems can leverage this through random walks in path planning, noisy activation functions in neural networks, random task allocation in swarm robotics, stochastic pheromone deposition, and mutation in evolutionary algorithms. Tuning the intensity of noise is crucial, and can be achieved through parameter optimization, adaptive noise injection, and experimental analysis. Future research focuses on theoretical understanding, adaptive noise control, hybrid approaches, and new applications. By embracing imperfection, Antetic AI can unlock new possibilities and achieve greater performance. Read more.
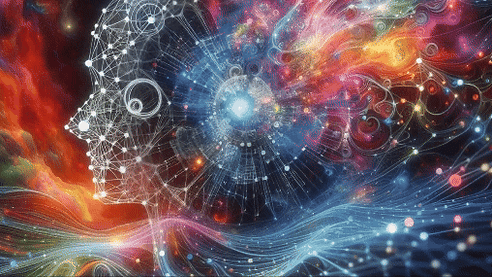_gif.gif)
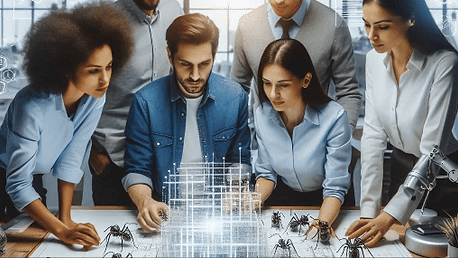_gif.gif)
Antetic AI distributes intelligence across interacting agents, requiring consideration of agent models, environment, communication mechanisms, task allocation, learning, and control. Architectural approaches include purely decentralized (high robustness, challenging to control), hierarchical (improved control, less robust), hybrid (balances benefits), environment-centric (maximizes stigmergy), and knowledge-based (improves efficiency in complex environments). The choice depends on task complexity, environmental dynamics, resource constraints, and design goals. Challenges involve formal design principles, creating tools and frameworks, exploring new approaches, developing performance metrics, and bridging the theory-practice gap. Architectural diversity is key for addressing diverse challenges. Read more.
Traditional agency, based on individual autonomy, intentionality, rationality, and responsibility, falters in Antetic AI where intelligence is distributed. There's a loss of centralized control, blurred intentionality, distributed responsibility, and emergent behavior. Reframing agency requires recognizing emergent agency, shared intentionality through environmental goals, distributed responsibility based on contribution, and accountability frameworks centered on system-level governance. Specific approaches include holistic system design, agent-level constraints with system-level oversight, transparency centered on collective outcomes, collective monitoring, and formal frameworks for distributed liability. This shift has implications for ethics, requiring frameworks focused on system-level impact, legal frameworks addressing collective responsibility, and public education centered on system-level understanding. Embracing collective action ensures Antetic AI is responsible and aligned with human values through ethically-informed system design. Read more.
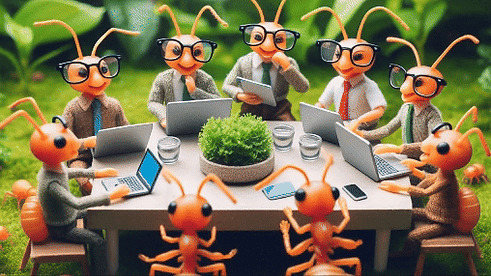_gif.gif)
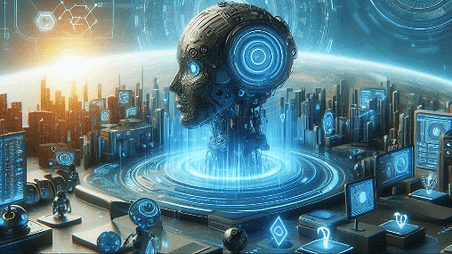_gif.gif)
Realizing sophisticated Antetic AI requires a specialized "Anthill Operating System" (Anthill OS) to address the limitations of general-purpose OSs (resource overhead, process management, communication infrastructure, real-time capabilities, lack of AI-specific abstractions). Anthill OS features a lightweight kernel, agent-centric design, scalable communication, real-time support, AI-specific abstractions, and hardware acceleration. Core components include a lightweight agent container, swarm communication layer, environmental interface, resource management system, AI services library, and security/isolation layer. Architectural considerations include microkernel vs. monolithic kernel, programming language choice, and hardware platform. Anthill OS improves performance, reduces resource consumption, simplifies development, enhances security, and provides greater flexibility. Challenges include developing a robust OS, maintaining compatibility, community adoption, integration with AI frameworks, and power-aware design. Read more.
"City Scavengers" proposes an Antetic AI ecosystem for proactive urban cleaning and maintenance, overcoming limitations of traditional methods through continuous, adaptive, and intelligent services. It comprises AI Ants (robotic agents with sensors, actuators, and behavioral rules), the Urban Environment (charging infrastructure, waste disposal, environmental sensors, a pheromone layer, and AR guidance), Anthill OS (orchestration engine for agent management, communication, data fusion, task allocation, and emergency coordination), and a Human Oversight System (monitoring dashboard, remote control, task prioritization, and data analysis). It operates through proactive exploration, stigmergic communication, dynamic task allocation, collaborative problem-solving, adaptive learning, and continuous monitoring. The benefits include improved cleanliness, reduced maintenance costs, increased efficiency, enhanced sustainability, and data-driven decision-making. Challenges include robustness, sensor accuracy, data security, ethical considerations, scalability, AI explainability, and integration with smart city initiatives. Read more.
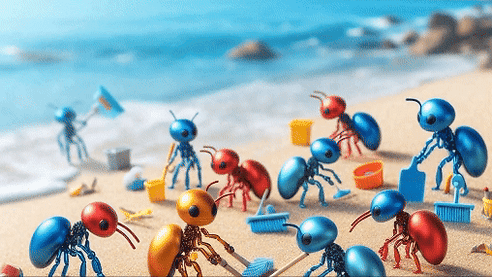_gif.gif)
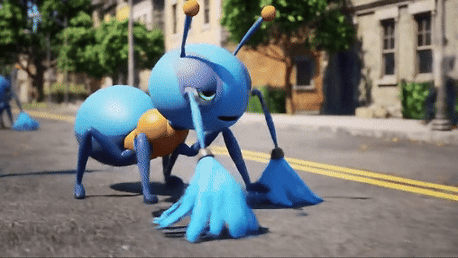_gif.gif)
"City Scavengers" concept, powered by Antetic AI and the Anthill OS, can be used to proactively address urban decay and prevent crime, drawing on the "broken windows theory." City Scavengers would use AI-powered "ants" to constantly monitor public spaces, promptly clean up litter and graffiti, and report infrastructure damage. This continuous maintenance signals care and order, deterring crime and fostering community pride. The article highlights the benefits of this approach, including efficiency, data-driven decision making, and avoiding potentially discriminatory policing practices. It also emphasizes the importance of integrating community engagement, like citizen reporting apps, and addresses challenges such as robot design, data privacy, and potential job displacement. Ultimately, City Scavengers aims to create a thriving urban ecosystem by fostering a shared commitment to maintaining and improving communities. Read more.
Antetic AI, a field inspired by self-assembly processes in ant colonies, aiming to create artificial systems that can autonomously construct complex structures. Self-assembly, where individual components organize without external direction, is contrasted with traditional manufacturing. Antetic AI mimics natural examples like protein folding and ant nest construction through decentralized control, local interactions, environmental sensing, self-organization, stigmergy, and fault tolerance. Mechanisms for self-assembly include shape-, chemical-, force-, energy-, and rule-based approaches. The article highlights applications in modular robotics, adaptive manufacturing, distributed sensor networks, and deployable structures for space exploration, including in-situ resource utilization. Challenges include design complexity, scalability, robustness, verification, and material properties. Future research directions involve developing sophisticated algorithms, exploring new materials, improving robustness, and integrating self-assembly with other AI techniques. The article concludes that self-assembly in Antetic AI holds significant potential for creating more adaptable, robust, and efficient systems in various fields. Read more.
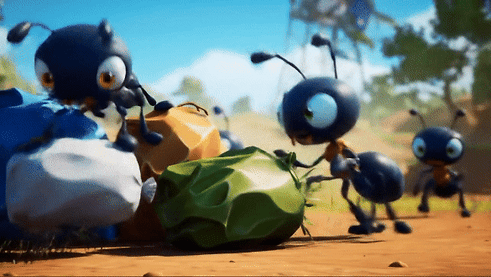_gif.gif)
Open Proposal: Pilot Program for City Scavengers - An Antetic AI Ecosystem for Proactive Urban Cleaning and Maintenance
This proposal invites forward-thinking governments to participate in a pilot program leveraging City Scavengers, an innovative urban cleaning and maintenance system powered by Antetic AI and the specialized Anthill OS.
Read more here and reach out to us by filling the form.